Groundbreaking Study Reveals Why Two-Stage AI Training Works Better Than Direct Optimization
This is a Plain English Papers summary of a research paper called Groundbreaking Study Reveals Why Two-Stage AI Training Works Better Than Direct Optimization. If you like these kinds of analysis, you should join AImodels.fyi or follow us on Twitter. Overview Research examines why two-stage fine-tuning (RM + RL) outperforms direct optimization Paper challenges intuition that two-stage processes should lose information Identifies "generation-verification gap" as key to explaining this discrepancy Finds that simpler reward models combined with RL-based policy search is more effective Results suggest RL's value comes from filtering policies that perform well for verifiers Plain English Explanation Why do the best AI language models use a seemingly roundabout training method? This paper tackles this puzzle. When experts fine-tune large language models like GPT-4, they typically use a two-step process. First, they train a "reward model" that learns human preferences. Then... Click here to read the full summary of this paper
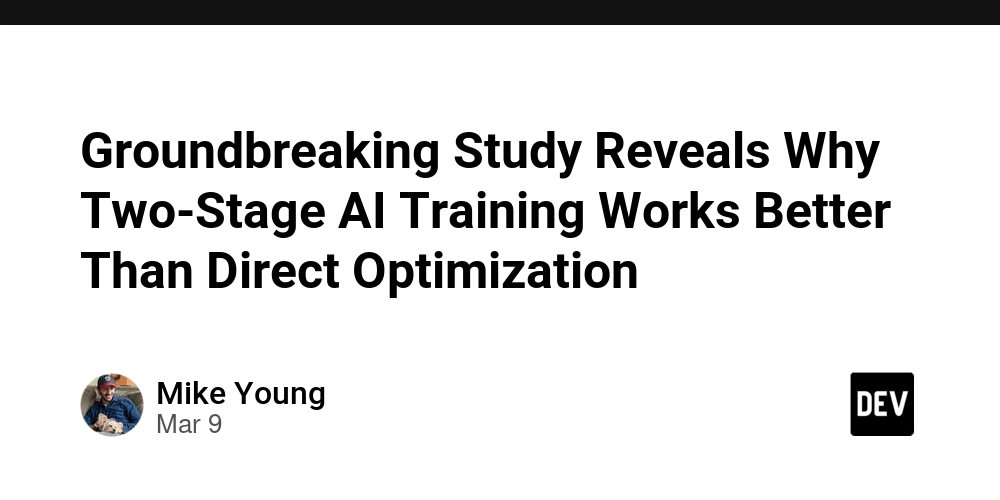
This is a Plain English Papers summary of a research paper called Groundbreaking Study Reveals Why Two-Stage AI Training Works Better Than Direct Optimization. If you like these kinds of analysis, you should join AImodels.fyi or follow us on Twitter.
Overview
- Research examines why two-stage fine-tuning (RM + RL) outperforms direct optimization
- Paper challenges intuition that two-stage processes should lose information
- Identifies "generation-verification gap" as key to explaining this discrepancy
- Finds that simpler reward models combined with RL-based policy search is more effective
- Results suggest RL's value comes from filtering policies that perform well for verifiers
Plain English Explanation
Why do the best AI language models use a seemingly roundabout training method? This paper tackles this puzzle.
When experts fine-tune large language models like GPT-4, they typically use a two-step process. First, they train a "reward model" that learns human preferences. Then...