Catch them Learning: A Pathway to Academic Integrity in the Age of AI
Clear, practical strategies for helping students use AI responsibly and maintain academic integrity. The post Catch them Learning: A Pathway to Academic Integrity in the Age of AI first appeared on Cult of Pedagogy.
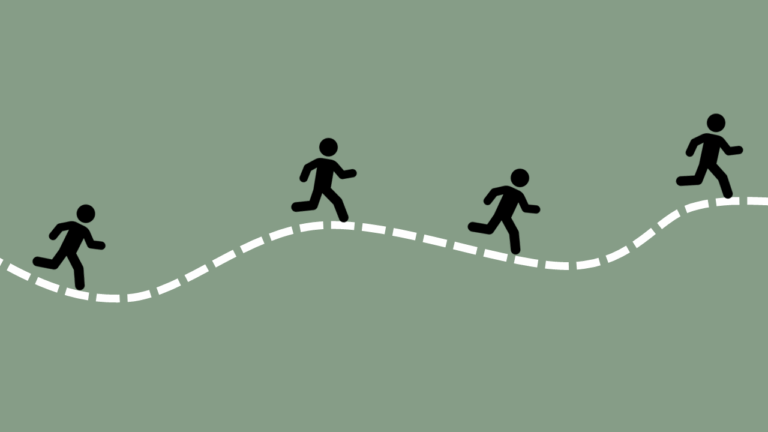
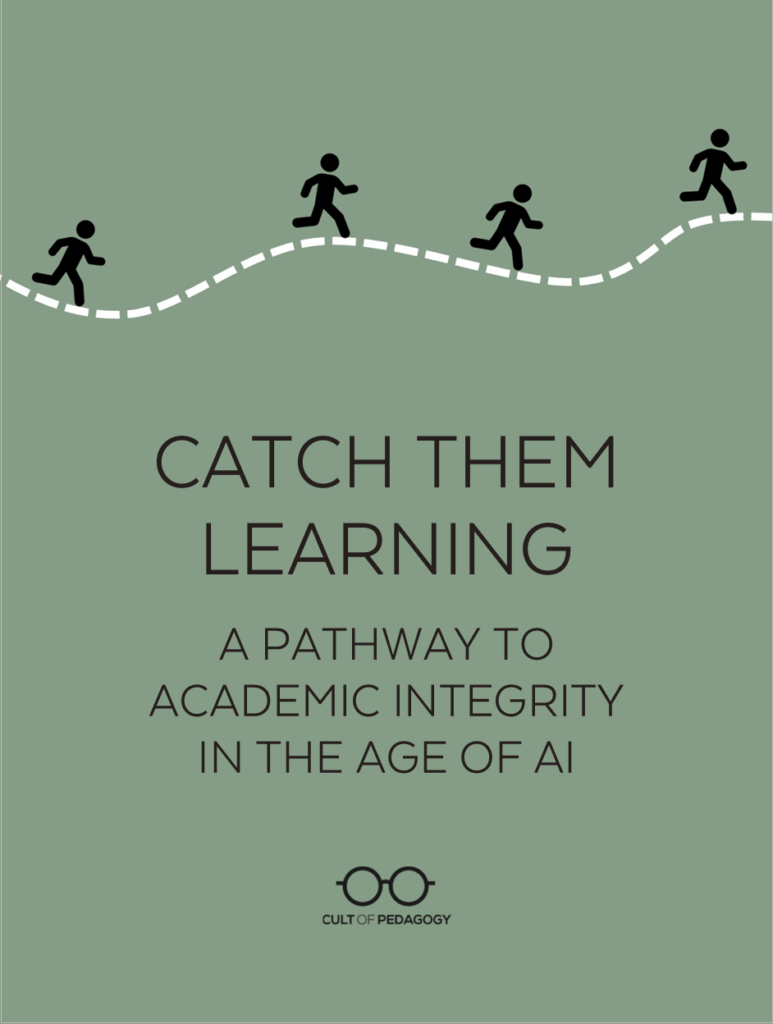
Listen to the interview with Tony Frontier:
Sponsored by ExploreLearning and Listenwise
This page contains Bookshop.org links. When you make a purchase through these links, Cult of Pedagogy gets a small percentage of the sale at no extra cost to you. What’s the difference between Amazon and Bookshop.org?
As the potential for students to misuse AI tools raises ongoing questions about accountability, cheating, and academic integrity, a scandal from the past offers insights into the future.
The Case of Rosie Ruiz
When Rosie Ruiz crossed the finish line of the 84th Boston Marathon, she raised her arms in triumph. Her winning time of 2:31:56 along the 26.2-mile course was one of the fastest ever recorded for a female marathoner.
Eight days later, Ruiz was formally accused of cheating in Boston and in a previous marathon. But because this was 1980, and the technologies that could be used to cheat were more advanced than the technology available to detect cheating, there was no definitive proof. The case reveals insights about a question relevant to a marathon course or an academic course: How can the integrity of results be ensured when there is easy access to technology that can be used to cheat?
Surprising Results
Ruiz’s victory in the women’s division of the Boston Marathon was a surprise. Several months earlier, she’d finished 11th in the New York City Marathon with a time of 2 hours and 56 minutes. A respectable time, but nowhere near her time in Boston. As usual, the winners of each division in Boston were interviewed just after the finish. A portion of Ruiz’s interview with noted marathoner and race announcer Katherine Switzer was as follows:
Switzer: “You improved from 2 hours and 56 minutes (in New York) to 2 hours and 31 minutes (today). What do you attribute that improvement in time to?”
Ruiz: “Um, I don’t know.”
Switzer: “Have you been doing a lot of heavy intervals?”
Ruiz: “Um, Someone else asked me that, I’m not sure what intervals are. What are they?”
Switzer: “Intervals are track workouts designed to make your speed improve dramatically…. Is someone coaching you or advising you?”
Ruiz: (chuckling) “Uh…No, I advise myself.”
Switzer: (looking directly into the camera) “…Rosie Ruiz. The mystery woman winner…we missed her at all our checkpoints….”
In the post-race press conference, Ruiz was baffled by more simple questions about her training methods, running jargon, race-day strategy, and observations along the route. Her inability to give specific answers raised suspicions about her results.
The Accusation, the Evidence, the Response
After an investigation involving police, race officials, and lawyers, Ruiz was disqualified. No images of Ruiz were found among 10,000 photos from the first 25 miles of the course. As she approached the finish, her speed was nowhere near the almost-world-record pace.
In the press conference after the disqualification, Ruiz vehemently denied any wrongdoing. Despite her promise to win again the next year, she never ran another marathon.
Missed Signals She’d Cheated in Her Previous Marathon
The press coverage from Boston caught the attention of a New Yorker named Susan Morrow. Morrow was surprised to read that Ruiz had finished in 11th place in the New York Marathon. Morrow had met Ruiz while she was riding the subway during that race. Ruiz told Morrow she’d injured her ankle early in the course and was taking the subway to the medical tent near the finish line. Morrow chatted with Ruiz along the entire 16-mile ride. Then, she and Ruiz exited the subway and watched the first female finishers complete the race. After that, she and Ruiz parted ways.
After Morrow shared her story, an investigation into Ruiz’s actions during the New York Marathon found that once she arrived at the medical tent, Ruiz told a race official she’d just finished, but no one had recorded her time. The official corrected the ‘mistake’ by writing down the time Ruiz had told him. The time put Ruiz in 11th place. If her sub-three-hour time had been legitimate, it would have been unbelievable: On her entry form, she estimated her completion time would be 4 hours and 10 minutes. Six months after the New York marathon had concluded, Ruiz was retroactively disqualified from that race.
The Motive
It is unknown whether Ruiz intended to cheat in New York or if the opportunity merely presented itself and she took it. What is known is that her colleagues at New York’s Metals Trading Institute were excited she was running the NYC Marathon and Ruiz had told them she expected to do well in the race. When she returned to work on the Monday after the race, her boss and colleagues greeted her as a hero. They were so impressed with her 11th place finish in New York that they chipped in to give her financial support to run the Boston Marathon. Perhaps, with their support, she could improve on her world-class time and even earn a spot on the podium? She didn’t disappoint. When she crossed the finish line in victory, she was wearing the “MTI” t-shirt they’d given her as a show of their pride for her to wear during the race.
Holding Students Accountable in an Era of AI
Whether it’s a subway along a marathon course or an AI tool in an academic course, technology can be misused to take shortcuts that render results meaningless. There are multiple pathways to holding students accountable for their evidence of learning; one is to focus on cheating, the other is to focus on integrity.
Integrity is often talked about as a coin that reads “cheated” on one side and “didn’t cheat” on the other. But integrity is a different coin entirely. Consider these definitions that I share in my book, AI with Intention: Principles and Action Steps for Teachers and School Leaders:
- Cheating is using a tool or resource to misrepresent one’s knowledge and skills to receive undue credit for a task. The accuser needs to support a claim about cheating with evidence that cheating occurred.
- Integrity describes a commitment to ensure one’s completion of a task accurately represents the knowledge and skills one actually possesses. A claim about integrity is supported by a learner’s ability to transparently document and reflect on the learning process and explain or expand on their results.
The Ruiz case is a cautionary tale about the limits of efforts to ensure accountability by focusing on cheating. Susan Morrow and Katherine Switzer revealed a different approach to accountability: the power of relationships, transparency, and explainability to ensure the integrity of results. The following steps can help you, and your students, take action to ensure academic integrity.
1. Acknowledge the limits of detecting and accusing students of cheating.
Data collected both before and after the release of ChatGPT show that about 65% of students reported that they’d cheated in the previous month (Lee et al., 2024); these rates are nearly identical to what students reported in previous years. In other words, regardless of students’ access to AI tools, cheating has been and continues to be endemic. The fact that AI detection tools are not foolproof (Fleckenstein et al., 2023) and can be gamed by students (Dougall, 2024), means accusations of cheating will continue to be difficult to prove, time-consuming, and require the teacher to carry the burden of accusatory proof.
The pressure students feel to get high grades — and the pressure for teachers to give them — are both real. Students know there will be consequences if they are caught cheating, but they weigh them against the consequences of not achieving. Meanwhile, teachers know that it can be difficult to prove cheating occurred, and there can be significant repercussions for a wrongful accusation.
If cheating is the claim, it must be proven by catching the cheater in the act, finding irrefutable evidence, or eliciting a confession. The Ruiz case is analogous to how this usually unfolds in the classroom: Irrefutable evidence is elusive, and confessions rarely happen. Even when caught in the act, students fall into a defensive mode similar to Ruiz; they bend the limits of logic and the laws of physics to deny any wrongdoing.
Many teachers have attempted to deter cheating with AI by threatening greater consequences. But increased consequences can have a paradoxical effect: The greater the consequences for the cheater, the greater the burden of proof for the accuser. This happened in Boston; minutes after Ruiz was crowned the winner, race officials doubted her surprising results. But the stakes of disqualifying the winner put officials in a state of paralysis; a formal investigation would be required before acknowledging any suspicious behavior.
In this era of easy-to-create but difficult-to-detect AI-generated text, many teachers find themselves in a similar state of paralysis. Absent foolproof detection tools, they feel powerless to do or say anything when they doubt a student’s results. But this paralysis is based on the false premise that only if AI detection tools are perfect can anything be done to prevent cheating or ensure integrity. By acknowledging the limits of strategies that seek to police integrity by catching students cheating, we can turn our attention to a more comprehensive set of strategies that minimize cheating and maximize integrity.
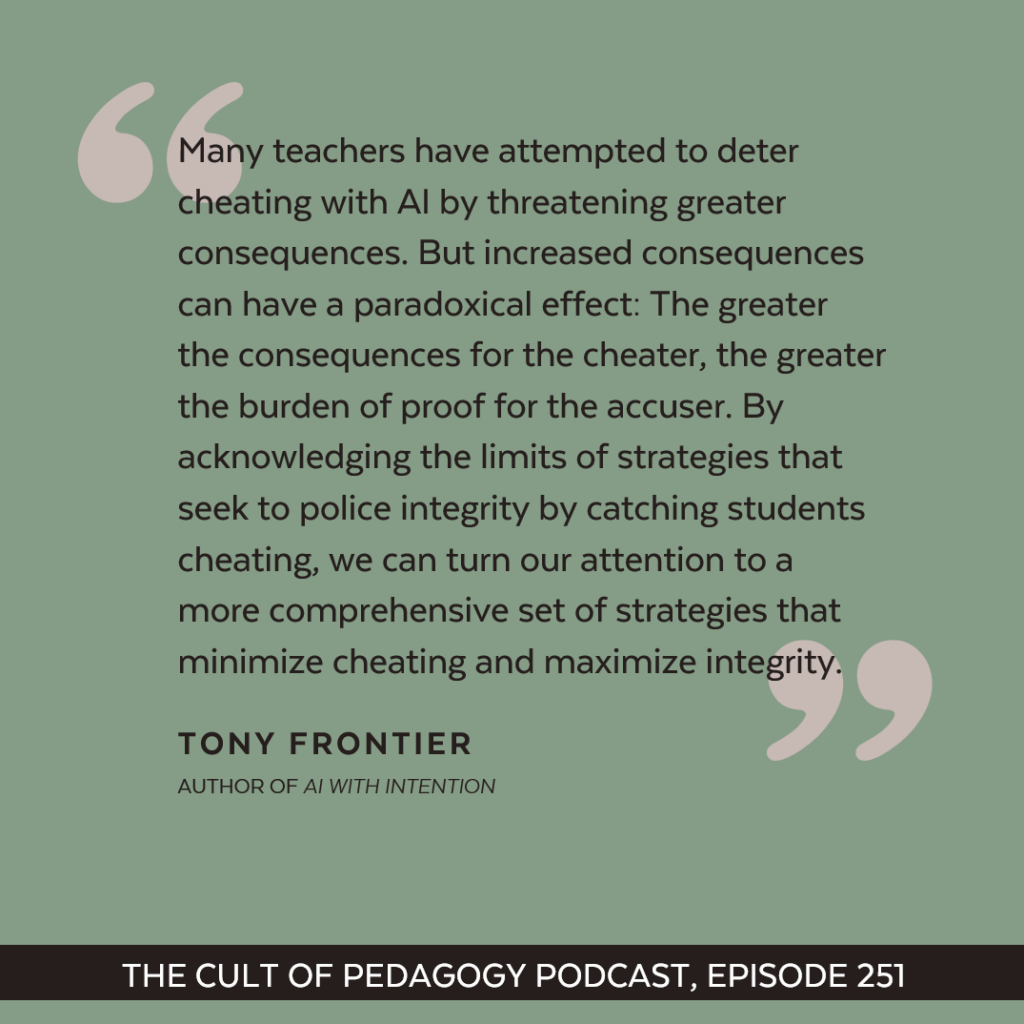
2. Minimize conditions where cheating is most likely to occur.
Addressing cheating primarily as deviant behavior that can be fixed with policies and punishments overlooks its root causes. When the pressure to achieve exceeds the fear of being caught, students easily rationalize why cheating is a logical, justifiable choice (Challenge Success, 2021). Consider the following classroom conditions associated with increased cheating:
- Students are more likely to cheat when they face unrealistic expectations to achieve. The company t-shirt Ruiz wore in Boston symbolized the unrealistic expectations she’d carried with her. High expectations are important for each learner; however, the weight of unrealistic expectations and the fear of disappointing others can easily exceed fears of being caught.
- Students are more likely to cheat when they believe they are anonymous. Ruiz learned in New York that amidst the crowds, race officials can’t keep track of individuals. When students don’t think their teachers know them well or don’t know what they are capable of academically, they are more likely to cheat.
- Students are more likely to cheat when they lack the time to produce quality results. Training for a marathon or developing skills and understandings in an academic course requires adherence to a months-long plan. Anyone who has worked with adolescents knows that planning and time management skills are challenging for students. When deadlines arrive earlier than anticipated, students look for shortcuts.
- Students are more likely to cheat when teachers emphasize performance goals rather than learning goals. In classrooms where teachers emphasize performance goals (errorless performance on tasks, points, and grades), students are more likely to cheat. (Miller, Murdock, Grotewiel, 2017).
When teachers are aware of and take action to minimize these factors, they can take action to minimize cheating.
3. Emphasize integrity by focusing on transparency, explainability, and relationships
Inquiry about cheating begins with the question “Did the student actually do the work?” Inquiry about integrity begins with the question “Does this work accurately represent the student’s skills and understandings?” Teachers can help learners support claims about the integrity of their work by consistently asking them to show evidence of transparency and explainability (Frontier, 2025).
- Transparency occurs when students document their steps and resources used to prepare for and engage in a task.
- Explainability occurs when students can explain, expand, and reflect on what they’ve learned and their evidence of learning.
What does this look like in practice? We can take some lessons from the Rosie Ruiz story.
Katherine Switzer didn’t accuse Ruiz of cheating in Boston. She simply asked Ruiz the questions she’d ask of any marathoner to transparently describe their training methods and explain what they saw and learned along the course. These questions weren’t accusations. In fact, for those who completed the entire course, they would be welcomed as expressions of interest. Ruiz’s inability to transparently reflect on her training or explain her results showed that her finishing time lacked integrity.
Similarly, Susan Morrow didn’t accuse Ruiz of cheating in New York. She was simply the only person who saw Ruiz as a unique individual on race day. No one else saw Ruiz as anything other than a number. Morrow’s interest in Ruiz lifted the veil of anonymity and allowed her to help hold Ruiz accountable.
These anecdotes can be transferred to specific strategies that support integrity, maximize learning, and minimize cheating in an academic course.
4. Use strategies that support integrity, maximize learning, and minimize cheating
As I argue in my book AI with Intention, if we want students to use AI tools with integrity, we must teach them how. Strategies that support integrity help students prioritize their efforts to engage in the learning process (Frontier, 2021), hold students accountable for evidence of important understandings (Wiggins & McTighe, 2006), and minimize the conditions where cheating is likely to occur (Miles et al., 2022).
4.1 Align expectations with opportunities to learn. The greater the alignment between stated teaching priorities and students’ learning opportunities, the better students can pursue rigorous and realistic goals.
- Share the map of the course in advance so students can see the important standards, learning goals, content, concepts, and skills.
- Communicate major assignments and assessments in advance and give students opportunities to plan their time and effort.
- Align formative tasks to summative goals to ensure students have had the opportunity to learn the content and skills required on projects and tasks.
- Use rubrics as a shared language of the route that teachers and students can use formatively to navigate the path toward high-quality work rather than as a summative tool to justify grades. For example, if you want students to “support claims with relevant details,” that expectation should be stated on a rubric that is given to students well in advance of when the assignment is due, and should be the focus of lessons, formative feedback, and student reflection.
- Focus on learning goals rather than errorless performance or task completion so students know it is okay to seek help and learn from mistakes. In a classroom focused on avoiding errors and completing tasks, students may see the purpose of a math assignment as completing items 1-17 without any errors so it can be checked and graded. You can shift this focus by establishing norms for asking clarifying questions, correcting errors, and reflecting on progress toward goals and mastery as the most important elements of the assignment.
4.2 Affirm students at the starting line. When students believe their teachers care about them and know their current abilities, they are more likely to act with integrity.
- Use an interest inventory to learn about students’ interests, aspirations, and preferred conditions for learning.
- Affirm personal interests by letting students know you cared enough to read their inventories. Students’ responses can be used to engage in conversations, inform the use of strategies, or make connections to course content.
- Gather baseline evidence of writing skills across academic disciplines using a simple, in-class prompt that requires students to provide a claim and supporting evidence in a full paragraph. This establishes a baseline of each student’s writing style, vocabulary, and skills.
- Affirm and provide feedback on (but don’t grade) baseline evidence so students know that you are aware of — and accept — their starting point. Quick feedback such as “I enjoyed reading your paragraph on (specific topic),” can be followed by an observation such as “Your use of well-aligned supporting details made this an interesting read.” Or, if more critical feedback is warranted, include a message of support, “I noticed some issues with your use of supporting details (see comments). Writing and reasoning get better with feedback and effort. I’m here to help!”
4.3 Create clear boundaries for what resources can, and cannot, be used for formative and summative work. Suppose race officials instituted a rule that said, “Any participant using the subway will be disqualified.” Does that include taking the subway to the starting line? To a running track for a training run? In an era of ubiquitous access to AI tools, simply saying “Using AI is cheating” can be interpreted by students to mean, “Well, then I guess everyone is cheating, so anything goes.”
- Clearly state boundaries for unacceptable use, so students know that copying or replicating answers from any source or tool without proper credit is cheating.
- State boundaries for acceptable use, so students know when it is acceptable to use AI tools to generate ideas, summarize information, engage in dialogue as a thought partner, receive feedback, etc.
- Teach strategies to use AI appropriately so students can use these tools as more than a mere shortcut. Students need to be taught how to use AI to engage in the learning process, make meaning of content, clarify misconceptions, and receive meaningful feedback. The potential of AI tools to be used in these ways is remarkable, but students need to be taught how to use them accordingly.
- Hold consistent, equitable expectations for transparency that require students to document and disclose their use of any resources, including AI tools, tutors, classmates, etc.
4.4 Acknowledge progress at checkpoints. Checkpoints along the route aren’t punitive. They ensure participants are on track toward a successful finish.
- Break major projects into smaller segments, steps, and deadlines so students can engage in the incremental process of planning, developing, revising, editing, and using feedback to refine their work.
- Do quick checks for understanding where students are required to complete and turn in brief, written responses to a problem or prompt in class. This could be an exit ticket related to an important learning goal or to glean insights into their efforts and progress on larger assignments.
- Teach students how to establish an appropriate pace by asking and expecting written responses to questions such as “The final phase of your project is due in a week: What have you accomplished? What are your next steps? What questions do you have?”
4.5 Provide consistent expectations for acknowledging sources and outside help. Arbitrary enforcement of policies confuses students. For example, an academic integrity policy might say “receiving outside assistance” is cheating, but students are rarely asked about assistance from parents, tutors, or apps. Or a policy might say “failure to properly cite sources” is plagiarism. However, students are often told to include specific information from assigned texts in their daily work, but they are rarely asked to acknowledge those texts as sources.
- Teach students how to cite sources. Give students examples of proper citation formatting — including for AI tools and textbooks — and expect students to use them throughout the school year. This could be done using formal or informal formatting.
- Teach students when to acknowledge others’ work or ideas. Citing sources is more than just proper formatting. Give students time to develop an understanding of when it is essential to acknowledge the ideas of others.
- Expect acknowledgment of sources throughout the course so students have formative opportunities to develop these skills on lower-stakes tasks throughout the year.
4.6 Consistently seek evidence of transparency and explainability. By asking questions like Katherine Switzer, you can help learners provide evidence of their effort, strategy, and understanding throughout the learning process.
- Gather evidence of transparency with prompts such as: (a) What process or strategies did you use to complete this assignment? (b) List any resources — including individuals, books, media, technology, and AI tools — you used for this assignment or assessment. (c) Attach any prompts and responses from AI tools with your completed work.
- Gather evidence of explainability by consistently asking questions such as: (a) Select an original quote from your writing that you are most proud of…Write the quote, and then explain your thinking in more detail. (b) Now that you’ve completed this assignment, what do you think are the three most important things to know about this topic and why? (c) Summarize your response/the most important ideas in your work in 50 words or less.
- Gather evidence of integrity as the norm, not the exception. If the only time we seek evidence of integrity is when a student is suspected of cheating, we’ve missed the mark. Prompts can be given as in-class pen-and-paper quick writes or posted in a content management system and completed during class time at different checkpoints and when an assignment is due.
- Ensure evidence of integrity before giving marks or grades. If a student turns in work that is significantly better (or different) than their evidence of explainability, it is not necessarily evidence of cheating. However, it is evidence that the student doesn’t understand the work they’ve done. This can serve as a catalyst to ask the student to re-engage in the original task to ensure their evidence reflects their actual understanding.
4.7 Frame integrity as the basis of your partnership for effective teaching and learning.
Regardless of your school’s academic integrity policy, consider how a statement like the one below in your syllabus (Frontier, 2025) could clarify the importance of integrity as the basis for your partnership to effectively teach and learn.
“It is important that you submit your own work so I can provide meaningful feedback to you to inform your next efforts to learn. If I don’t know what you do or don’t know, I can’t adjust my instruction to better support your learning. It’s okay not to know. It’s okay to ask questions. If you knew all of this already, there’d be no need for you to take this class.
Academic integrity means you own what you know, acknowledge what you don’t know, and are transparent about the ideas or words you use that were drawn from others’ work or through the use of AI tools. Sometimes, I’ll ask you to retrace your steps so I can affirm – or assist with – the process you’ve used to complete a task. I’ll always ask you to cite your sources. I’ll always expect you to give credit to others or to a technology tool when credit is due.
Academic dishonesty involves any attempt to take credit for knowledge or skills that you don’t actually possess as your own. If you cannot explain your work after it has been completed, it may or may not be evidence of academic dishonesty. However, it is evidence that you haven’t internalized that knowledge or those skills yet. If that is the case, I need to know so I can help you take the next steps necessary to learn.”
The Path Forward
Today’s students can easily access AI tools to take shortcuts through any academic course. By using strategies that support integrity, transparency, and explainability, we can work in partnership with our students to help them use tools and resources in ways that ensure we’ll have plenty of opportunities to catch them learning and celebrate their results. And when they step up to the microphone after they’ve run their best race, they’ll be able to speak with confidence and pride about the strategies they used, what they learned about important content and skills, and what they learned about themselves.
References
Challenge Success (2021). Cheat or be cheated? What we know about academic integrity. Retrieved from: https://challengesuccess.org/resources/cheat-or-be-cheated-what-we-know-about-academic-integrity
Dougall, J. (2024). My AI experiment: how many students can beat detection software? Tes Magazine. https://www.tes.com/magazine/teaching-learning/secondary/how-easy-is-it-to-cheat-with-AI-without-being-detected-in-school
Fleckenstein, J., Meyer, J., Jansen, T., Keller, S. D., Köller, O., & Möller, J. (2024). Do teachers spot AI? Evaluating the detectability of AI-generated texts among student essays. Computers and Education: Artificial Intelligence, 6, 100209. https://doi.org/10.1016/j.caeai.2024.100209
Frontier, T. (2021). Teaching with clarity: How to prioritize and do less so students understand more. ASCD.
Frontier, T. (2025). AI with intention: Principles and action steps for teachers and school leaders. ASCD.
Lee, V.R., Pope, D., Miles, S., & Zárate, R.C. (2024). Cheating in the age of generative AI: A high school survey study of cheating behaviors before and after the release of ChatGPT. Computers and Education: Artificial Intelligence, v7:1-10.
Miles, P., Campbell, M., Ruxton, G. (2022). Why Students Cheat and How Understanding This Can Help Reduce the Frequency of Academic Misconduct in Higher Education: A Literature Review. Journal of Undergraduate Neuroscience Education. 20(2), 150-160.
Miller, A., Murdock, T., & Grotewiel, M. (2017). Addressing Academic Dishonesty Among the Highest Achievers. Theory Into Practice. 56:1-8. 10.1080/00405841.2017.1283574.
Wiggins, G., & McTighe, J. (2006). Understanding by Design. ASCD.
Tony Frontier, PhD, is a researcher, author, and consultant. His latest book, AI with Integrity: Principles and Action Steps for Teachers and School Leaders will be published by ASCD in May of 2025. He can be reached through LinkedIn, his website, or by email at tonyfrontier@gmail.com.
Join our mailing list and get weekly tips, tools, and inspiration that will make your teaching more effective and fun. You’ll get access to our members-only library of free downloads, including 20 Ways to Cut Your Grading Time in Half, the e-booklet that has helped thousands of teachers save time on grading. Over 50,000 teachers have already joined—come on in.