The Future of Search: A Proposal for Reliable Information Retrieval
A deep dive into how AI is reshaping search, the risks we face, and a potential path forward We all know what it means, colloquially, to google something. For decades, we've been typing keywords into search boxes, getting back blue links, and clicking through to find what we need. But as MIT Technology Review points out, "all that is up for grabs. We are at a new inflection point." And what an inflection point it is. The Evolution of Search: From Keywords to AI's Conversational Future The story of search engines is a tale of constant evolution. In the beginning, there was Archie - the first real internet search engine that crawled through files hidden in the darkness of remote servers. It didn't tell you what was in those files - just their names. Then came Yahoo's hierarchical directory, a human-curated attempt to organize the chaos of the early web. Enter Google in 1998, revolutionizing search by looking not just at content, but at the sources linking to a website. The more something was cited elsewhere, the more reliable Google considered it. This breakthrough made Google radically better at retrieving relevant results than anything that had come before. But now we're entering an era of what Google's CEO Sundar Pichai describes as "one of the most positive changes we've done to search in a long, long time" - conversational AI search. Instead of keywords, you use real questions expressed in natural language. But Google isn't alone in this revolution. OpenAI's ChatGPT now has access to the web, Microsoft has integrated generative search into Bing, and players like Perplexity are pushing the boundaries with their own approaches. The goal? To move from keyword-based searching to conversational interactions that provide direct answers written by generative AI based on live information from across the internet rather than just links. The Dead Internet Theory and the AI Content Flood Here's where things get interesting - and potentially terrifying. Enter the "Dead Internet Theory," a concept that asserts that since 2016 or 2017, the internet has consisted mainly of bot activity and automatically generated content manipulated by algorithmic curation to control the population and minimize organic human activity. While this might sound like a conspiracy theory, recent developments in AI are making parts of it feel uncomfortably plausible. Consider OpenAI's latest creation, Operator, their new AI agent that can, as described in their announcement, "use its own browser to perform tasks for you. […] it's a research preview of an agent that can use the same interfaces and tools that humans interact with on a daily basis." This development, while impressive, raises an unsettling question: What happens when the internet becomes a playground for AI agents talking to other AI agents? The situation becomes even more complex when we consider that many Large Language Models (LLMs) are trained on internet data. As Patrick Lewis, the lead author of the 2020 paper that coined the term RAG (Retrieval-Augmented Generation), points out, these models can't always be trusted. "When it doesn't have an answer, an AI model can blithely and confidently spew back a response anyway," causing what Google's chief scientist for search, Pandu Nayak, Google's chief scientist for search, calls "a real problem" for everyone. The AI-Generated Content Crisis The issue runs deeper than just unreliable answers. We're facing what could be called an "AI content flood." As MIT Technology Review reveals, when Google began testing AI-generated responses to search queries, things didn't go well. "Google, long the world's reference desk, told people to eat rocks and to put glue on their pizza," an incident that highlighted the fundamental challenges of AI-generated content. But perhaps the greatest hazard lies in what the industry calls "the loop" - AI systems training on content generated by other AI systems. As the Dead Internet Theory suggests, we might be heading toward a future where most online content is AI-generated, creating a dangerous feedback loop where AI systems learn from increasingly artificial and potentially unreliable information. "You're always dealing in percentages," says Google CEO Sundar Pichai about AI accuracy. "I'd say 99-point-few-nines. I think that's the bar we operate at, and it is true with AI Overviews too." But when every AI system is learning from other AI systems' outputs, those few errors could compound exponentially. This recursive problem demands a solution that can ground AI responses in reliable, verified information rather than potentially AI-generated content. RAG: A Light in the Dark This is where Retrieval-Augmented Generation (RAG) enters the picture, a promising approach that directly addresses this challenge. While traditional AI models risk perpetuating a cycle of artificial content, RAG breaks this loop by anchoring AI responses to reliable external sources. As explained by AWS, RAG is a technique for enhan
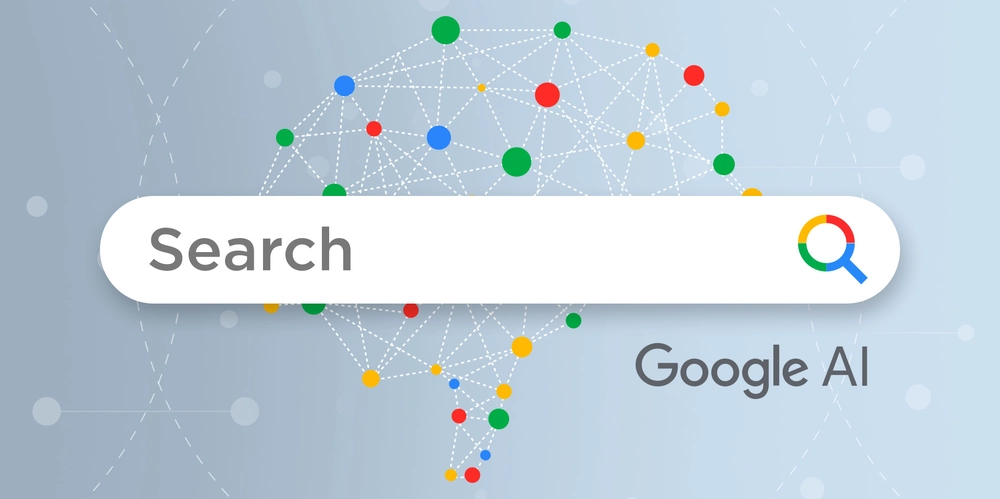
A deep dive into how AI is reshaping search, the risks we face, and a potential path forward
We all know what it means, colloquially, to google something. For decades, we've been typing keywords into search boxes, getting back blue links, and clicking through to find what we need. But as MIT Technology Review points out, "all that is up for grabs. We are at a new inflection point." And what an inflection point it is.
The Evolution of Search: From Keywords to AI's Conversational Future
The story of search engines is a tale of constant evolution. In the beginning, there was Archie - the first real internet search engine that crawled through files hidden in the darkness of remote servers. It didn't tell you what was in those files - just their names. Then came Yahoo's hierarchical directory, a human-curated attempt to organize the chaos of the early web.
Enter Google in 1998, revolutionizing search by looking not just at content, but at the sources linking to a website. The more something was cited elsewhere, the more reliable Google considered it. This breakthrough made Google radically better at retrieving relevant results than anything that had come before.
But now we're entering an era of what Google's CEO Sundar Pichai describes as "one of the most positive changes we've done to search in a long, long time" - conversational AI search. Instead of keywords, you use real questions expressed in natural language. But Google isn't alone in this revolution. OpenAI's ChatGPT now has access to the web, Microsoft has integrated generative search into Bing, and players like Perplexity are pushing the boundaries with their own approaches. The goal? To move from keyword-based searching to conversational interactions that provide direct answers written by generative AI based on live information from across the internet rather than just links.
The Dead Internet Theory and the AI Content Flood
Here's where things get interesting - and potentially terrifying. Enter the "Dead Internet Theory," a concept that asserts that since 2016 or 2017, the internet has consisted mainly of bot activity and automatically generated content manipulated by algorithmic curation to control the population and minimize organic human activity. While this might sound like a conspiracy theory, recent developments in AI are making parts of it feel uncomfortably plausible.
Consider OpenAI's latest creation, Operator, their new AI agent that can, as described in their announcement, "use its own browser to perform tasks for you. […] it's a research preview of an agent that can use the same interfaces and tools that humans interact with on a daily basis." This development, while impressive, raises an unsettling question: What happens when the internet becomes a playground for AI agents talking to other AI agents?
The situation becomes even more complex when we consider that many Large Language Models (LLMs) are trained on internet data. As Patrick Lewis, the lead author of the 2020 paper that coined the term RAG (Retrieval-Augmented Generation), points out, these models can't always be trusted. "When it doesn't have an answer, an AI model can blithely and confidently spew back a response anyway," causing what Google's chief scientist for search, Pandu Nayak, Google's chief scientist for search, calls "a real problem" for everyone.
The AI-Generated Content Crisis
The issue runs deeper than just unreliable answers. We're facing what could be called an "AI content flood." As MIT Technology Review reveals, when Google began testing AI-generated responses to search queries, things didn't go well. "Google, long the world's reference desk, told people to eat rocks and to put glue on their pizza," an incident that highlighted the fundamental challenges of AI-generated content.
But perhaps the greatest hazard lies in what the industry calls "the loop" - AI systems training on content generated by other AI systems. As the Dead Internet Theory suggests, we might be heading toward a future where most online content is AI-generated, creating a dangerous feedback loop where AI systems learn from increasingly artificial and potentially unreliable information.
"You're always dealing in percentages," says Google CEO Sundar Pichai about AI accuracy. "I'd say 99-point-few-nines. I think that's the bar we operate at, and it is true with AI Overviews too." But when every AI system is learning from other AI systems' outputs, those few errors could compound exponentially. This recursive problem demands a solution that can ground AI responses in reliable, verified information rather than potentially AI-generated content.
RAG: A Light in the Dark
This is where Retrieval-Augmented Generation (RAG) enters the picture, a promising approach that directly addresses this challenge. While traditional AI models risk perpetuating a cycle of artificial content, RAG breaks this loop by anchoring AI responses to reliable external sources. As explained by AWS, RAG is a technique for enhancing the accuracy and reliability of generative AI models by referencing authoritative knowledge bases outside their training data. Think of it as giving an AI system a reliable library to check its facts against before speaking.
Unlike traditional AI models that rely solely on their training data, RAG allows systems to actively retrieve and verify information from trusted sources before generating responses. It's the difference between a student writing an essay purely from memory versus one who consults peer-reviewed sources while writing.
A Proposal for the Future of Search
Here's where we get to the meat of the matter: what if we combined the conversational capabilities of modern AI with RAG's ability to verify information against trusted sources? Imagine a search engine that could:
Understand your questions in natural language
Search through a curated database of verified, reliable sources
Generate comprehensive answers while citing its sources
Maintain accuracy without sacrificing the convenience of direct answers
The initial database could include:
Peer-reviewed scientific journals
Academic publications
Verified news sources
Government databases
Professional industry publications
Expert-validated technical documentation
This approach would combine the best of both worlds: the accuracy and reliability of traditional search with the convenience and natural interaction of AI systems. As NVIDIA's technical brief explains, RAG allows us to maintain "accuracy, appropriateness and security" while still leveraging the power of large language models.
The Thorny Questions
However, this proposal raises some challenging questions. First, who gets to decide what sources are considered "reliable"? There's a risk of creating what could essentially become a digital gatekeeping system, potentially limiting the democratizing nature of the internet.
Furthermore, as the AWS points out, RAG systems currently face technical limitations. They require significant computational resources to process large amounts of data quickly enough for real-time search applications. There's also the challenge of keeping the trusted database current in a world where information changes rapidly.
Looking Ahead: The Technical and Ethical Horizon
For this vision to become reality, several technical advances are needed:
More efficient RAG systems capable of processing massive amounts of data in real-time
Better vector databases for storing and retrieving information
More sophisticated natural language understanding to better match queries with relevant sources
Improved fact-checking and validation mechanisms
But perhaps the most crucial challenge lies in finding the right balance between accessibility and reliability. As Nick Turley of OpenAI points out in their Operator announcement, "Having a model in the loop is a very, very different mechanism than how a search engine worked in the past." We need to ensure that in our quest to make information more accessible, we don't inadvertently create new forms of digital divide or information gatekeeping.
Conclusion: A Path Forward
The future of search doesn't have to be a choice between the wild west of AI-generated content and the cumbersome process of manual research. By combining RAG with carefully curated information sources, we might find a middle path that preserves the benefits of AI while maintaining the reliability we need.
Will it be perfect? No. As Google's Pandu Nayak reminds us, you're always dealing in percentages. But it could be significantly better than either our current search paradigm or an AI-only future. The key lies not in choosing between human and artificial intelligence, but in finding ways to let each complement the other's strengths while mitigating their respective weaknesses.
The internet doesn't have to die. It just needs to evolve - thoughtfully, carefully, and with a clear eye on both the potential and the pitfalls ahead.