The Intersection of Neuroscience and AI in John Ball’s Work
Explore how John Ball bridges neuroscience and AI, creating innovative models that mimic human cognition and advance the future of machine intelligence.
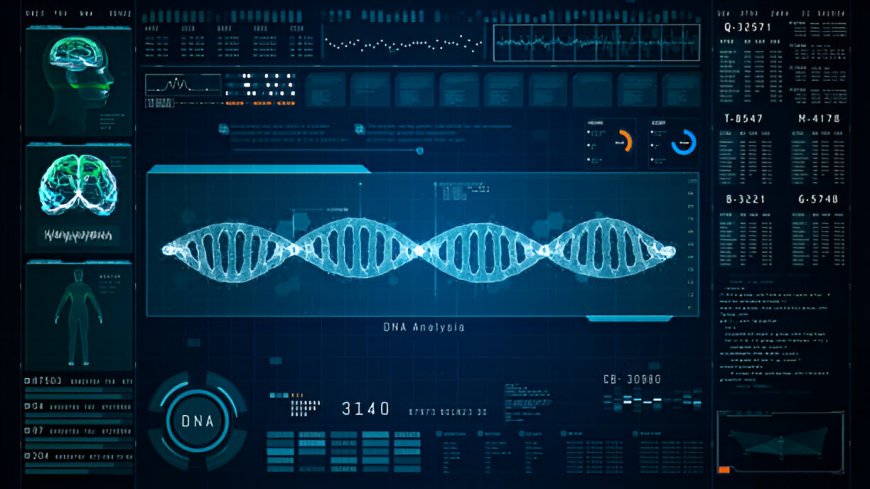
Bridging Biology and Computation
The ever-evolving field of artificial intelligence (AI) has long drawn from neuroscience for inspiration. From the earliest days of neural networks to today’s explorations in cognitive architectures, researchers have sought to understand and replicate the brain’s inner workings. Few have approached this intersection with the depth and consistency of John Ball. His work not only underscores the significance of neuroscience in AI but also presents a compelling framework for machine intelligence that mimics human thought processes more closely than traditional models. Ball’s approach stands out for its focus on meaning, context, and human-like understanding, placing him at the forefront of a more nuanced AI future.
A Brain-Based Approach to AI
Ball’s dedication to integrating neuroscience into AI stems from a deep belief that genuine intelligence must reflect how the human brain processes information. Unlike many AI researchers who focus primarily on statistical or probabilistic models, Ball examines how neurons connect ideas, memories, and emotions. This biological realism sets his work apart, especially when considering how human beings use contextual understanding to make decisions. By grounding his theories in the human brain's structure and behavior, Ball offers a refreshing and vital direction in the quest for artificial general intelligence (AGI).
A key representation of this integration is his Machine Intelligence Book By John Ball , which explores the profound relationship between cognitive science and intelligent systems. Rather than creating systems that only simulate intelligence through predictive algorithms, Ball dives into how machines can think—not just process. In the , he maps cognitive functions to algorithmic processes, bringing in insights from brain regions such as the prefrontal cortex, hippocampus, and amygdala. These references aren't abstract; they are central to his models of machine reasoning and memory. His argument is clear: without mirroring the brain’s organizational principles, AI will remain limited to narrow, task-specific capabilities.
Meaning and Context in Language Understanding
One of Ball’s most notable contributions is his Meaning-Based Natural Language Understanding (MBNLU) framework. This model draws heavily from neuroscience, particularly how humans associate meaning with words based on prior experience and context. In typical AI systems, language understanding often revolves around token frequencies and vector similarities. Ball challenges this approach. He argues that true understanding must come from the relational structure of knowledge—how neurons associate seemingly unrelated experiences through meaning, emotional value, and learned patterns. This emphasis on meaning, drawn from neuroscience, enables a far more flexible and adaptive AI framework.
Neural Mappings and Mental States
Ball’s fascination with the brain is not merely philosophical—it’s also methodological. He emphasizes the idea of “neural mappings” where individual units represent concepts, emotions, or experiences. This mirrors how the human brain uses synaptic connections to encode complex mental states. His work often references how the hippocampus processes memory consolidation or how the prefrontal cortex manages executive function. These biological processes, he believes, are essential to achieving machines that can reason, plan, and adapt like humans. By mapping AI processes to these neural mechanisms, Ball opens a path toward systems that can genuinely interact with the world as humans do.
The Role of Emotions in Intelligence
Another critical area of Ball’s work involves emotional cognition. Traditional AI systems either ignore emotion or treat it as a superficial layer for interaction. Ball, drawing from neuroscience, suggests that emotions are integral to decision-making. In the human brain, emotions guide reasoning, bias perception, and shape memory. He argues that an intelligent machine without emotional modeling is inherently incomplete. Through his research, he explores how artificial systems can integrate emotional feedback into decision processes—mirroring the way our brains evaluate risk, reward, and ethical concerns.
Brain-Inspired Architectures in AI
Furthermore, Ball advocates for a brain-inspired architecture in building AI systems. This means not only simulating neuron-like functions but structuring machines around key brain functions. For example, he explores how working memory can be replicated in machines by implementing temporary knowledge buffers, similar to the brain's dorsolateral prefrontal cortex. He also studies long-term memory formation through reinforcement, akin to how humans consolidate knowledge over time. These concepts are not just technical proposals; they reflect a philosophy of AI that deeply respects biological intelligence.
Consciousness and Explainability
What separates Ball from many in the AI space is his insistence on explainability and consciousness. Drawing from neuroscience, he contends that consciousness is not a mystical attribute but a product of layered awareness and feedback loops in the brain. If we can understand how the brain constructs awareness—through constant monitoring, error correction, and self-representation—then we can begin designing systems that simulate conscious behavior. This perspective pushes AI beyond mechanical computation toward something that could understand itself and its actions.
Bridging Disciplinary Gaps
His interdisciplinary approach also attracts attention in academic and industrial circles. Neuroscientists appreciate how Ball respects the brain’s complexity, while AI researchers find practical guidance in his cognitive models. His work serves as a bridge between fields often isolated by jargon and specialization. More importantly, he encourages a new generation of AI designers to study neuroscience—not as a side interest, but as a central tenet of building intelligent systems.
A Timely Contribution to Modern AI
Ball's contributions are particularly timely as society questions the limits of current AI systems. With growing concern over hallucinations in large language models and the lack of common-sense reasoning in many bots, his neuroscience-rooted ideas offer hope. He advocates for meaning-first design, context-aware memory, and emotionally grounded processing. These aren’t just idealistic goals; they are grounded in decades of neuroscience research and translated into working prototypes in Ball’s lab.
The Future of Human-Centered AI
The real strength of Ball’s work lies in its ability to rethink AI’s foundations. By placing neuroscience at the center, he helps shift AI away from brute-force learning toward elegant, efficient intelligence. His models are not just more explainable; they are inherently more human. As we look ahead to a future where machines interact more deeply with our lives, Ball’s insistence on biological realism could ensure that AI systems understand our needs, values, and experiences more genuinely.
Moreover, Ball’s influence is beginning to shape educational approaches. Several universities are now integrating his research into their AI and neuroscience curricula. They recognize that tomorrow’s AI engineers must think biologically, philosophically, and computationally. This shift reflects a broader movement in the tech world, where interdisciplinary thinking is becoming the gold standard for innovation.
Conclusion
In conclusion, John Ball’s work at the intersection of neuroscience and AI offers a groundbreaking reimagining of what intelligent machines can become. His commitment to biological realism, emotional cognition, and contextual reasoning paves the way for a new era in artificial intelligence—one that doesn’t just mimic human output but emulates human thought. As the boundaries between brain science and machine learning continue to blur, Ball’s insights remain not only relevant but essential. His legacy will likely influence AI development for decades to come, anchoring the field in a deeper understanding of how we, as humans, think, feel, and know.