Why Data Is the Unsung Hero of AI Strategy
The AI Gold Rush – From Pilots and Experiments to Enterprise Scale and Strategy Moore’s Law is well and truly in play when it comes to AI. AI is heavily in demand, and every enterprise is adopting AI. Innovation is also helping fuel this demand with new AI models, AI Agents, and new technologies coming […] The post Why Data Is the Unsung Hero of AI Strategy appeared first on Unite.AI.
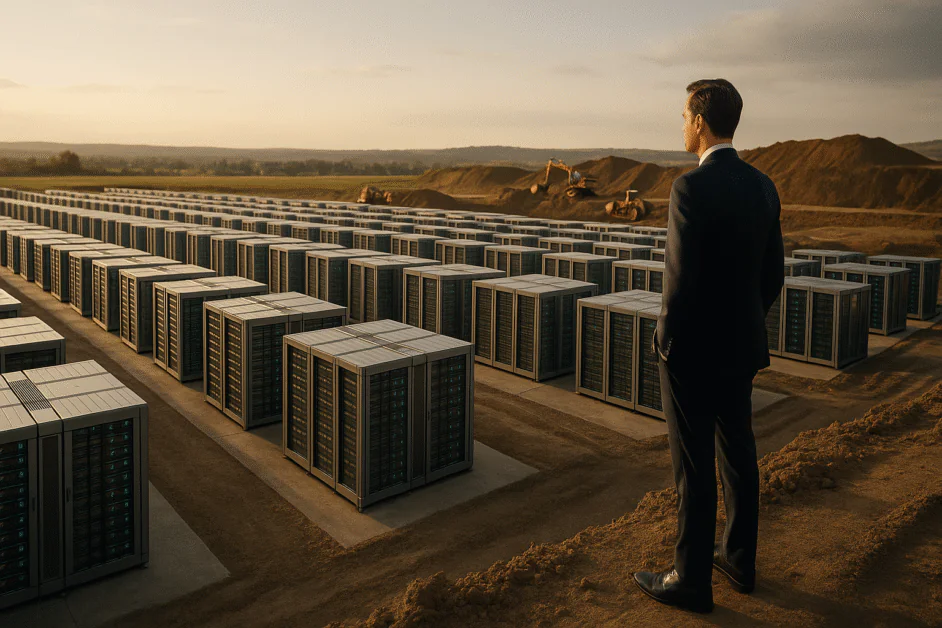
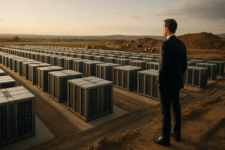
The AI Gold Rush – From Pilots and Experiments to Enterprise Scale and Strategy
Moore’s Law is well and truly in play when it comes to AI. AI is heavily in demand, and every enterprise is adopting AI. Innovation is also helping fuel this demand with new AI models, AI Agents, and new technologies coming into this place. This is creating a fundamental shift for enterprises – the stage for pilots and cool experiments and showcases for AI, in particular, Generative AI is largely fading. Enterprises are realizing that AI needs to be embedded as part of the Enterprise strategy for scaling and creating true business differentiation. AI is a topic in most boardrooms, resulting in strategic innovation and budgets.
Data: The First Domino in AI Strategy
A key consideration in any AI strategy should be Data. Data is critical for AI models to be contextual, intelligent, and domain and enterprise-specific. AI models predict outcomes based on both the way the model is tuned and the inputs presented to it. Both of these depend on the quality, variety, recency, and structure of the data.
According to a recent IDC forecast, AI is expected to boost the global economy by nearly $20 trillion by 2030, driven not only by models but also by massive investments in the underlying data and infrastructure that fuel them.
Training data with narrow subsets leads to biased models, outdated data leads to irrelevant results, and poor data just leads to poor AI results. Therefore, Data is the first domino in an enterprise’s data strategy. Even with the best people and cutting-edge technologies, if the data domino falls, the entire AI strategy tumbles down quickly.
As Gartner’s 2024 report on top data and analytics trends notes, organizations as they scale with AI depend on data, and the leaders who succeed will be those who establish trust in their data and lead with it strategically.
Key Strategic Data Decisions for your AI Strategy
Here are 5 key considerations you and your enterprise need to make for on preparing your Data for your AI strategy:
1. Reuse your Data landscape – Several enterprises don’t reuse the data management, data governance, and data storage and analytics landscape for AI. A lot of data serving critical reporting and analytics can also be critical for AI. It is therefore important to start with the data assets already present in the enterprise. Of course, this needs to be augmented with the right data quality measures.
Key Question to Ask – What data do we have in our enterprise, and what condition is it in?
2. Metadata and Data Lineage – For the data in place, metadata, i.e., data about the data, might be just as critical, if not more, for AI. For instance, the business terms tagged to the data can help identify the relevant context for a RAG model, for instance. When a user asks for the status of a claim in an Insurance enterprise, all the data attributes tagged with Claim status can be used as context for the AI model to respond. Data Lineage also helps understand the flow of the data, helping the AI models to identify trusted data sources.
Based on a recent ISASA blog, AI Governance is critical and requires the right metadata and data lineage to scale.
Key Question to Ask – Is our data tagged properly with business and technical metadata? Do we collect data lineage to understand how the data flows end to end?
3. Data Governance and Compliance – Ensure that your data is well governed and managed, and that any compliance and privacy regulations are applied to the data. The AI Strategy should then inherit and extend these governance and regulations than starting from scratch. For instance, if a customer wants their data to be anonymized as per GDPR regulations, an AI model should be both trained and operational on the anonymized dataset.
Key Question to Ask – Do we have a Data Governance and Compliance program in place? If not, what are the key aspects that I need to have in place for my AI strategy?
4. Treat Master Data as your AI Quarterback – Critical Master Data, which contains data about the key entities in your enterprise, should be used as the base for your AI strategy. For instance, if the 360 degree view of a customer exists, an AI strategy on any customer domain, such as a customer churn prediction, should leverage this master data to avoid any data missed or incomplete. Of course, this can be combined with more information from specific data sources.
Key Question to Ask – Do I have my critical master data domains available in a complete and connected to the rest of my data landscape?
5. Data and its value – Data should not be treated as a cost center but measured in terms of its value, both towards AI and the business. This requires data to be on Board and CXO topics in addition to AI.
Key Question to Ask – Does my Board and CXOs understand the value of Data to the organization? If not, how can we ensure that this is understood, especially in the context of the AI strategy in the enterprise?
Models Come and Go, But Data Endures.
As your AI strategy evolves, new models and AI innovations will emerge. The speed of innovation in this space is mind-boggling. But over time, AI models will commoditize; the true differentiator in your enterprise is not which model you use but how it gets contextualized with what data is training, fine-tuning, and working on it.
If you’re crafting an AI strategy, don’t start with the model. Start with the question: Do we have the data to support it?
The post Why Data Is the Unsung Hero of AI Strategy appeared first on Unite.AI.