Partitioning in Distributed Data Systems: Explained with Real-World Examples
When systems grow beyond a single machine's capabilities, partitioning (also called sharding) becomes essential. Chapter 6 of "Designing Data-Intensive Applications" by Martin Kleppmann dives deep into how partitioning works and the challenges it introduces. Let's break it down into an easy-to-understand tech blog, complete with practical examples! Why Partition Data? Partitioning distributes data across multiple nodes to: Scale storage beyond a single machine. Improve query throughput and reduce latency. Increase system fault tolerance. Without partitioning, a database might hit bottlenecks in CPU, RAM, disk, or network. Real-World Example: Twitter stores billions of tweets. A single server can't handle this load, so tweets are partitioned based on user IDs across many servers. Strategies for Partitioning Data 1. Key Range Partitioning Data is partitioned based on a continuous range of keys. Example: Users with IDs 0-1000 are stored on Server A. Users with IDs 1001-2000 are stored on Server B. Pros: Efficient range queries. Cons: Hotspots can occur if many accesses are skewed toward certain ranges (e.g., famous users). 2. Hash Partitioning Apply a hash function to a key to determine the partition. Example: hash(user_id) % 4 decides one of four servers. Pros: More even distribution of data. Cons: Range queries become inefficient. 3. Directory-Based Partitioning Maintain a lookup service that maps each key to its partition. Example: A metadata service keeps track of which shard holds which user's data. Pros: Flexibility to rebalance partitions easily. Cons: Extra overhead and complexity in managing the directory. Challenges of Partitioning 1. Uneven Data Distribution (Skew) Some partitions grow larger than others. Real-World Example: In a photo-sharing app, a celebrity's account might have millions of photos, causing their partition to grow disproportionately. Solutions: Careful choice of partition key. Dynamic rebalancing. 2. Rebalancing Partitions As data grows, you might need to move data from one node to another. Problem: Moving data is expensive and can impact performance. Solutions: Use consistent hashing. Implement automatic load balancing. 3. Transactions Across Partitions Transactions spanning multiple partitions are complex and slower. Real-World Example: Transferring money between two users stored in different partitions. Solutions: Use distributed transaction protocols like two-phase commit (2PC). Or, design systems to avoid multi-partition transactions if possible. 4. Partitioning Secondary Indexes Not just the main data, but indexes must be partitioned too. Challenge: Queries on secondary attributes (like "find users by email") might require broadcasting queries across partitions. Real-World Problems: Suppose you shard your database by user_id, but you want to find a user by their email. The email lookup must search across all partitions unless a secondary index exists. Solutions: Local Secondary Indexes: Each partition maintains an index for only its own data. Efficient but queries might need to touch multiple partitions. Global Secondary Indexes: Build a separate distributed service that indexes attributes like email globally across all partitions. Requires careful consistency management. Denormalization: Store redundant information alongside the main record to avoid secondary lookups. Best Practice: Evaluate which queries are most frequent. Create targeted secondary indexes accordingly. Beware of consistency trade-offs in global indexes! Example: Amazon DynamoDB allows you to define both local and global secondary indexes depending on your query patterns. Partitioning and Query Execution A key challenge is routing a query to the correct partition: With good partitioning, queries hit a single node. Bad partitioning might need scatter-gather: query all nodes and aggregate results. Real-World Example: Amazon Dynamo uses partition awareness to direct reads/writes to the right server efficiently. Trade-Off Table for Partitioning Strategies Strategy Pros Cons Best Use Case Key Range Partitioning Great for range queries Hotspots under skewed loads Time-series data, sequential IDs Hash Partitioning Even load distribution Poor for range queries High-velocity user-generated content (e.g., tweets) Directory-Based Partitioning Flexible, rebalancing-friendly Directory service overhead Dynamic, evolving data models Final Thoughts Partitioning is powerful but intricate. Choosing the right partitioning strategy and key is critical to ensure that the system scales well, maintains performance, and avoids hotspots. Understanding these principles helps you design systems that can scale effortlessly and serve millio
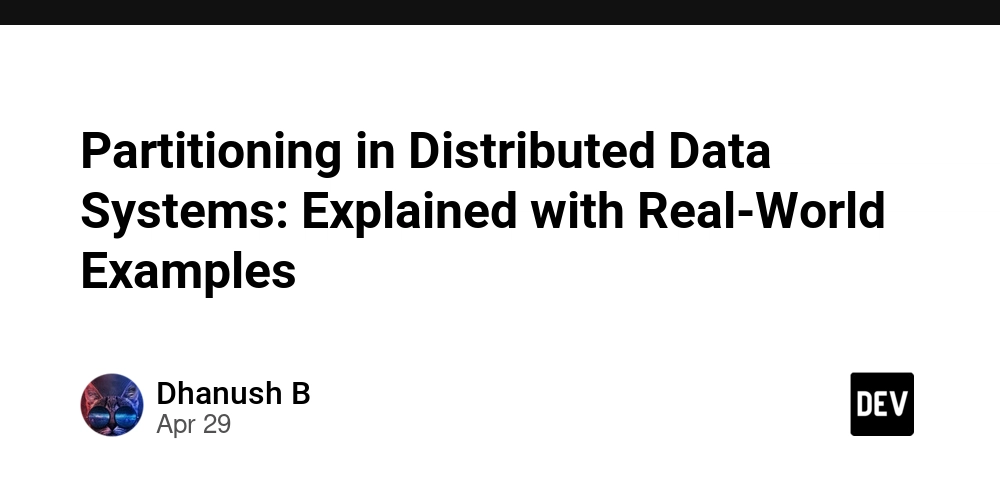
When systems grow beyond a single machine's capabilities, partitioning (also called sharding) becomes essential. Chapter 6 of "Designing Data-Intensive Applications" by Martin Kleppmann dives deep into how partitioning works and the challenges it introduces. Let's break it down into an easy-to-understand tech blog, complete with practical examples!
Why Partition Data?
Partitioning distributes data across multiple nodes to:
- Scale storage beyond a single machine.
- Improve query throughput and reduce latency.
- Increase system fault tolerance.
Without partitioning, a database might hit bottlenecks in CPU, RAM, disk, or network.
Real-World Example:
- Twitter stores billions of tweets. A single server can't handle this load, so tweets are partitioned based on user IDs across many servers.
Strategies for Partitioning Data
1. Key Range Partitioning
- Data is partitioned based on a continuous range of keys.
Example:
- Users with IDs 0-1000 are stored on Server A.
- Users with IDs 1001-2000 are stored on Server B.
Pros:
- Efficient range queries.
Cons:
- Hotspots can occur if many accesses are skewed toward certain ranges (e.g., famous users).
2. Hash Partitioning
- Apply a hash function to a key to determine the partition.
Example:
-
hash(user_id) % 4
decides one of four servers.
Pros:
- More even distribution of data.
Cons:
- Range queries become inefficient.
3. Directory-Based Partitioning
- Maintain a lookup service that maps each key to its partition.
Example:
- A metadata service keeps track of which shard holds which user's data.
Pros:
- Flexibility to rebalance partitions easily.
Cons:
- Extra overhead and complexity in managing the directory.
Challenges of Partitioning
1. Uneven Data Distribution (Skew)
- Some partitions grow larger than others.
Real-World Example:
- In a photo-sharing app, a celebrity's account might have millions of photos, causing their partition to grow disproportionately.
Solutions:
- Careful choice of partition key.
- Dynamic rebalancing.
2. Rebalancing Partitions
- As data grows, you might need to move data from one node to another.
Problem:
- Moving data is expensive and can impact performance.
Solutions:
- Use consistent hashing.
- Implement automatic load balancing.
3. Transactions Across Partitions
- Transactions spanning multiple partitions are complex and slower.
Real-World Example:
- Transferring money between two users stored in different partitions.
Solutions:
- Use distributed transaction protocols like two-phase commit (2PC).
- Or, design systems to avoid multi-partition transactions if possible.
4. Partitioning Secondary Indexes
- Not just the main data, but indexes must be partitioned too.
Challenge:
- Queries on secondary attributes (like "find users by email") might require broadcasting queries across partitions.
Real-World Problems:
- Suppose you shard your database by
user_id
, but you want to find a user by theiremail
. The email lookup must search across all partitions unless a secondary index exists.
Solutions:
- Local Secondary Indexes: Each partition maintains an index for only its own data. Efficient but queries might need to touch multiple partitions.
- Global Secondary Indexes: Build a separate distributed service that indexes attributes like email globally across all partitions. Requires careful consistency management.
- Denormalization: Store redundant information alongside the main record to avoid secondary lookups.
Best Practice:
- Evaluate which queries are most frequent.
- Create targeted secondary indexes accordingly.
- Beware of consistency trade-offs in global indexes!
Example:
- Amazon DynamoDB allows you to define both local and global secondary indexes depending on your query patterns.
Partitioning and Query Execution
A key challenge is routing a query to the correct partition:
- With good partitioning, queries hit a single node.
- Bad partitioning might need scatter-gather: query all nodes and aggregate results.
Real-World Example:
- Amazon Dynamo uses partition awareness to direct reads/writes to the right server efficiently.
Trade-Off Table for Partitioning Strategies
Strategy | Pros | Cons | Best Use Case |
---|---|---|---|
Key Range Partitioning | Great for range queries | Hotspots under skewed loads | Time-series data, sequential IDs |
Hash Partitioning | Even load distribution | Poor for range queries | High-velocity user-generated content (e.g., tweets) |
Directory-Based Partitioning | Flexible, rebalancing-friendly | Directory service overhead | Dynamic, evolving data models |
Final Thoughts
Partitioning is powerful but intricate. Choosing the right partitioning strategy and key is critical to ensure that the system scales well, maintains performance, and avoids hotspots.
Understanding these principles helps you design systems that can scale effortlessly and serve millions or billions of users without breaking a sweat.
Next time you're designing a backend, remember: how you cut your data shapes everything that follows!
Inspired by "Designing Data-Intensive Applications" by Martin Kleppmann.