Core Principles of HTM
Hierarchical Temporal Memory (HTM) is a theoretical framework for understanding the neocortex, the part of the brain responsible for perception, movement, and intelligence. Developed by Jeff Hawkins and his team at Numenta, HTM models aim to replicate the structural and algorithmic properties of the neocortex to create systems that learn and behave in ways similar to biological brains. Core Principles of HTM Sparse Distributed Representations (SDRs): HTM utilizes SDRs to represent information. In these representations, only a small percentage of neurons are active at any given time, leading to efficient and noise-resistant data encoding. Temporal Sequence Learning: HTM networks are designed to learn and predict sequences of patterns over time, capturing temporal dependencies and enabling the anticipation of future inputs based on historical data. Hierarchical Organization: Mirroring the structure of the neocortex, HTM is organized hierarchically, allowing for the processing of information at varying levels of abstraction. Applications of HTM Anomaly Detection: HTM has been effectively applied in identifying anomalies in streaming data, such as monitoring server logs or financial transactions, by learning normal patterns and detecting deviations. Sensorimotor Integration: Research is ongoing into using HTM for robotics, where the integration of sensory inputs with motor actions can lead to more adaptive and intelligent behaviors. Challenges and Future Directions While HTM offers a biologically inspired approach to machine intelligence, it faces challenges in scalability and integration with existing machine learning frameworks. Ongoing research aims to address these issues and explore the potential of HTM in various domains, including artificial intelligence and neuroscience. In summary, Hierarchical Temporal Memory provides a compelling model for understanding and replicating the functions of the neocortex, with promising applications in anomaly detection and beyond..read more
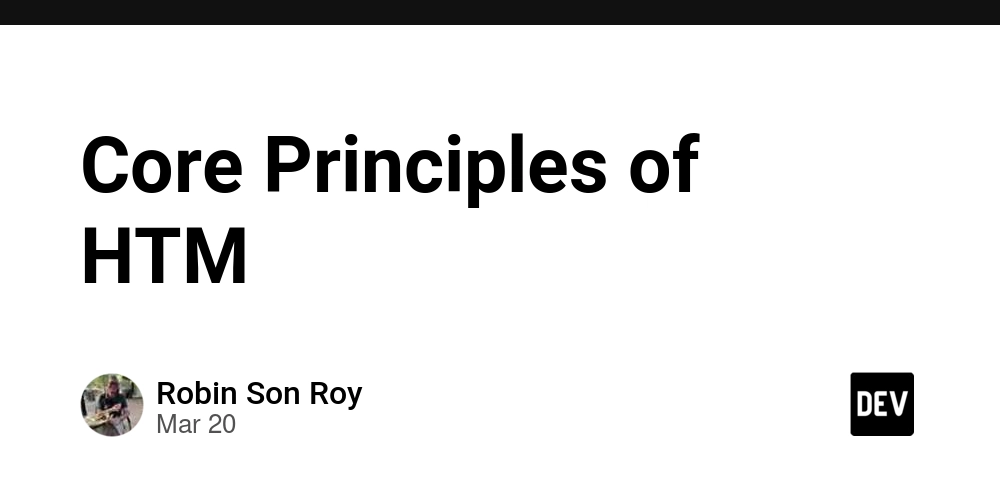
Hierarchical Temporal Memory (HTM) is a theoretical framework for understanding the neocortex, the part of the brain responsible for perception, movement, and intelligence. Developed by Jeff Hawkins and his team at Numenta, HTM models aim to replicate the structural and algorithmic properties of the neocortex to create systems that learn and behave in ways similar to biological brains.
Core Principles of HTM
Sparse Distributed Representations (SDRs):
HTM utilizes SDRs to represent information. In these representations, only a small percentage of neurons are active at any given time, leading to efficient and noise-resistant data encoding.
Temporal Sequence Learning:
HTM networks are designed to learn and predict sequences of patterns over time, capturing temporal dependencies and enabling the anticipation of future inputs based on historical data.
Hierarchical Organization:
Mirroring the structure of the neocortex, HTM is organized hierarchically, allowing for the processing of information at varying levels of abstraction.
Applications of HTM
Anomaly Detection:
HTM has been effectively applied in identifying anomalies in streaming data, such as monitoring server logs or financial transactions, by learning normal patterns and detecting deviations.
Sensorimotor Integration:
Research is ongoing into using HTM for robotics, where the integration of sensory inputs with motor actions can lead to more adaptive and intelligent behaviors.
Challenges and Future Directions
While HTM offers a biologically inspired approach to machine intelligence, it faces challenges in scalability and integration with existing machine learning frameworks. Ongoing research aims to address these issues and explore the potential of HTM in various domains, including artificial intelligence and neuroscience.
In summary, Hierarchical Temporal Memory provides a compelling model for understanding and replicating the functions of the neocortex, with promising applications in anomaly detection and beyond..read more