The Man Out to Prove How Dumb AI Still Is
François Chollet has constructed the ultimate test for the bots.
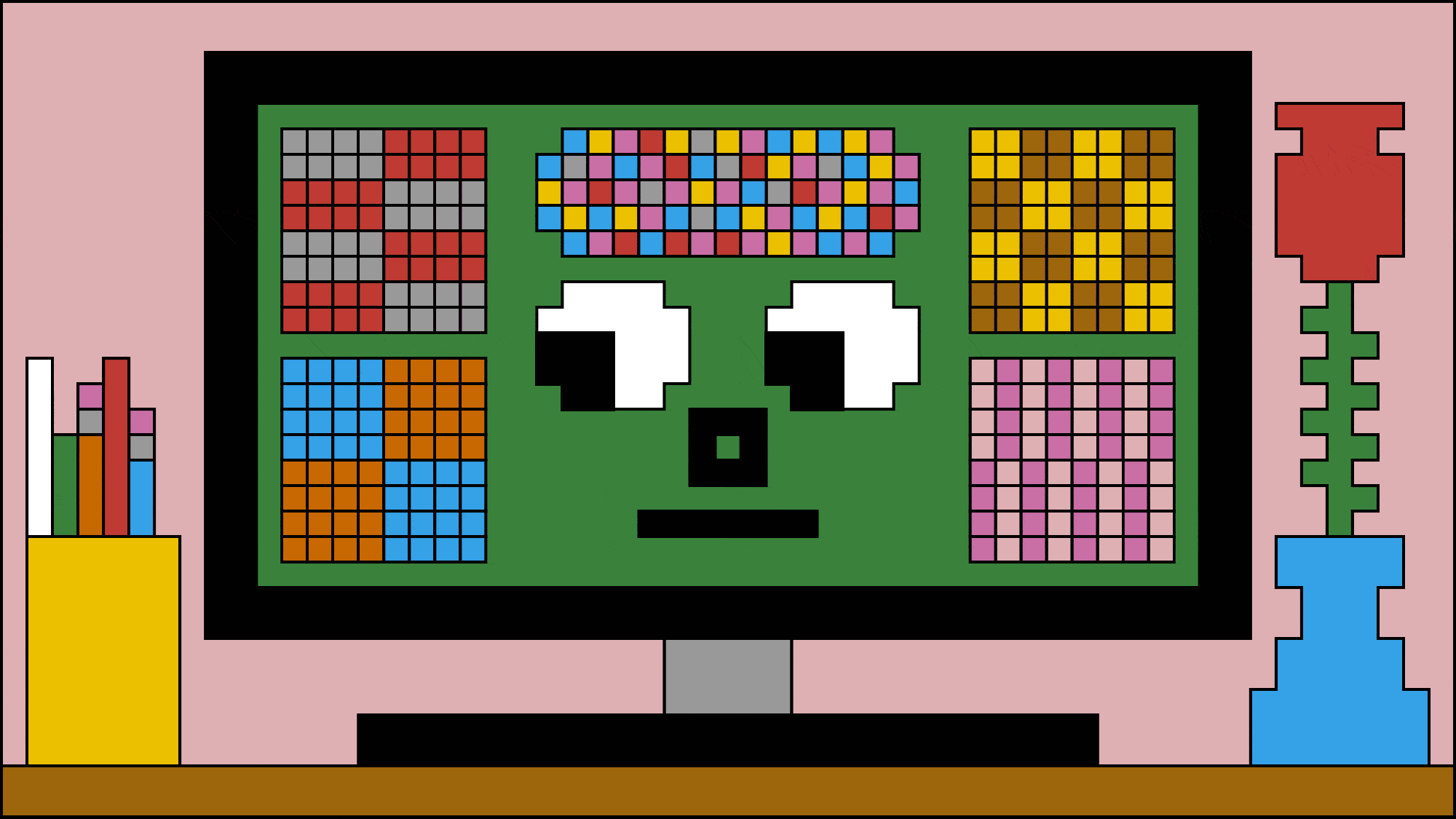
Deep down, Sam Altman and François Chollet share the same dream. They want to build AI models that achieve “artificial general intelligence,” or AGI—matching or exceeding the capabilities of the human mind. The difference between these two men is that Altman has suggested that his company, OpenAI, has practically built the technology already. Chollet, a French computer scientist and one of the industry’s sharpest skeptics, has said that notion is “absolutely clown shoes.”
When I spoke with him earlier this year, Chollet told me that AI companies have long been “intellectually lazy” in suggesting that their machines are on the path to a kind of supreme knowledge. At this point, those claims are based largely on the programs’ ability to pass specific tests (such as the LSAT, Advanced Placement Biology, and even an introductory sommelier exam). Chatbots may be impressive. But in Chollet’s reckoning, they’re not genuinely intelligent.
Chollet, like Altman and other tech barons, envisions AI models that can solve any problem imaginable: disease, climate change, poverty, interstellar travel. A bot needn’t be remotely “intelligent” to do your job. But for the technology to fulfill even a fraction of the industry’s aspirations—to become a researcher “akin to Einstein,” as Chollet put it to me—AI models must move beyond imitating basic tasks, or even assembling complex research reports, and display some ingenuity.
Chollet isn’t just a critic, nor is he an uncompromising one. He has substantial experience with AI development and created a now-prominent test to gauge whether machines can do this type of thinking. For years, he has contributed major research to the field of deep learning, including at Google, where he worked as a software engineer from 2015 until this past November; he wants generative AI to be revolutionary, but worries that the industry has strayed. In 2019, Chollet created the Abstraction and Reasoning Corpus for Artificial General Intelligence, or ARC-AGI—an exam designed to show the gulf between AI models’ memorized answers and the “fluid intelligence” that people have. Drawing from cognitive science, Chollet described such intelligence as the ability to quickly acquire skills and solve unfamiliar problems from first principles, rather than just memorizing enormous amounts of training data and regurgitating information. (Last year, he launched the ARC Prize, a competition to beat his benchmark with a $1 million prize fund.)
You, a human, would likely pass this exam. But for years, chatbots had a miserable time with it. Most people, despite having never encountered ARC-AGI before, get scores of roughly 60 to 70 percent. GPT-3, the program that became ChatGPT, the legendary, reality-distorting bot, scored a zero. Only recently have the bots started to catch up.
How could such powerful tools fail the test so spectacularly for so long? This is where Chollet’s definition of intelligence comes in. To him, a chatbot that has analyzed zillions of SAT-style questions, legal briefs, and lines of code is not smart so much as well prepared—for the SAT, a law-school exam, advanced coding problems, whatever. A child figuring out tricky word problems after just learning how to multiply and divide, meanwhile, is smart.
ARC-AGI is simple, but it demands a keen sense of perception and, in some sense, judgment. It consists of a series of incomplete grids that the test-taker must color in based on the rules they deduce from a few examples; one might, for instance, see a sequence of images and observe that a blue tile is always surrounded by orange tiles, then complete the next picture accordingly. It’s not so different from paint by numbers.
The test has long seemed intractable to major AI companies. GPT-4, which OpenAI boasted in 2023 had “advanced reasoning capabilities,” didn’t do much better than the zero percent earned by its predecessor. A year later, GPT-4o, which the start-up marketed as displaying “text, reasoning, and coding intelligence,” achieved only 5 percent. Gemini 1.5 and Claude 3.7, flagship models from Google and Anthropic, achieved 5 and 14 percent, respectively. These models may have gotten lucky on a few puzzles, but to Chollet they hadn’t evinced a shred of abstract reasoning. “If you were not intelligent, like the entire GPT series,” he told me, “you would score basically zero.” In his view, the tech barons were not even on the right path to building their artificial Einstein.
[Read: The GPT era is already ending]
Chollet designed the grids to be highly distinctive, so that similar puzzles or relevant information couldn’t inadvertently be included in a model’s training data—a common problem with AI benchmarks. A test taker must start anew with each puzzle, applying basic notions of counting and geometry. Most other AI evaluations and standardized tests are crude by comparison—they aren’t designed to evaluate a distinct, qualitative aspect of thinking. But ARC-AGI checks for the ability to “take concepts you know and apply them to new situations very efficiently,” Melanie Mitchell, an AI researcher at the Santa Fe Institute, told me.
To improve their performance, Silicon Valley needed to change its approach. Scaling AI—building bigger models with more computing power and more training data—clearly wasn’t helping. OpenAI was first to market with a model that even came close to the right kind of problem-solving. The firm announced a so-called reasoning model, o1, this past fall that Altman later called “the smartest model in the world.” Mark Chen, OpenAI’s chief research officer, told me the program represented a “new paradigm.” The model was designed to check and revise its approach to any question and to spend more time on harder ones, as a human might. An early version of o1 scored 18 percent on ARC-AGI—a definite improvement, but still well below human performance. A later iteration of o1 hit 32 percent. OpenAI was still “a long way off” from fluid intelligence, Chollet told me in September.
That was about to change. In late December, OpenAI previewed a more advanced reasoning model, o3, that scored a shocking 87 percent on ARC-AGI—making it the first AI to match human performance on the test and the best-performing model by far. Chollet described the program as a “genuine breakthrough.” o3 appeared able to combine different strategies on the fly, precisely the kind of adaptation and experimentation needed to succeed on ARC-AGI.
Unbeknownst to Chollet, OpenAI had kept track of his test “for quite a while,” Chen told me in January. Chen praised the “genius of ARC,” calling its resistance to memorized answers a good “way to test generalization, which we see as closely linked to reasoning.” And as the start-up’s reasoning models kept improving, ARC-AGI resurfaced as a meaningful challenge—so much so that the ARC Prize team collaborated with OpenAI for o3’s announcement, during which Altman congratulated them on “making such a great benchmark.”
Chollet, for his part, told me he feels “pretty vindicated.” Major AI labs were adopting, even standardizing, his years-old ideas about fluid intelligence. It is not enough for AI models to memorize information: They must reason and adapt. Companies “say they have no interest in the benchmark, because they are bad at it,” Chollet said. “The moment they’re good at it, they will love it.”
Many AI proponents were quick to declare victory when o3 passed Chollet’s test. “AGI has been achieved in 2024,” one start-up founder wrote on X. Altman wrote in a blog post that “we are now confident we know how to build AGI as we have traditionally understood it.” Since then, Google, Anthropic, xAI, and DeepSeek have launched their own “reasoning” models, and the CEO of Anthropic, Dario Amodei, has said that artificial general intelligence could arrive within a couple of years.
But Chollet, ever the skeptic, wasn’t sold. Sure, AGI might be getting closer, he told me—but only in the sense that it had previously been “infinitely” far away. And just as this hurdle was cleared, he decided to raise another.
Last week, the ARC Prize team released an updated test, called ARC-AGI-2, and it appears to have sent the AIs back to the drawing board. The full o3 model has not yet been tested, but a version of o1 dropped from 32 percent on the original puzzles to just 3 percent on the new version, and a “mini” version of o3 currently available to the public dropped from roughly 30 percent to below 2 percent. (An OpenAI spokesperson declined to say whether the company plans to run the benchmark with o3.) Other flagship models from OpenAI, Anthropic, and Google have achieved roughly 1 percent, if not lower. Human testers average about 60 percent.
If ARC-AGI-1 was a binary test for whether a model had any fluid intelligence, Chollet told me last month, the second version aims to measure just how savvy an AI is. Chollet has been designing these new puzzles since 2022; they are, in essence, much harder versions of the originals. Many of the answers to ARC-AGI were immediately recognizable to humans, while on ARC-AGI-2, people took an average of five minutes to find the solution. Chollet believes the way to get better on ARC-AGI-2 is to be smarter, not to study harder—a challenge that may help push the AI industry to new breakthroughs. He is turning the ARC Prize into a nonprofit dedicated to designing new benchmarks to guide the technology’s progress, and is already working on ARC-AGI-3.
[Read: DOGE’s plans to replace humans with AI are already under way]
Reasoning models take bizarre and inhuman approaches to solving these grids, and increased “thinking” time will come at substantial cost. To hit 87 percent on the original ARC-AGI test, o3 spent roughly 14 minutes per puzzle and, by my calculations, may have required hundreds of thousands of dollars in computing and electricity; the bot came up with more than 1,000 possible answers per grid before selecting a final submission. Mitchell, the AI researcher, said this approach suggests some degree of trial and error rather than efficient, abstract reasoning. Chollet views this inefficiency as a fatal flaw, but corporate AI labs do not. If chatbots achieve fluid intelligence in this way, it will not be because the technology approximates the human mind: You can’t just stuff more brain cells into a person’s skull, but you can give a chatbot more computer chips.
In the meantime, OpenAI is “shifting towards evaluations that reflect utility as well,” Chen told me, such as tests of an AI model’s ability to navigate and take actions on the web—which will help the company make better, although not necessarily smarter, products. OpenAI itself, not some third-party test, will ultimately decide when its products are useful, how to price them (perhaps $20,000 a year for a “Phd-level” bot, according to one report), and whether they’ve achieved AGI. Indeed, the company may already have its own key AGI metric, of a sort: As The Information reported late last year, Microsoft and OpenAI have come to an agreement defining AGI as software capable of generating roughly $100 billion in profits. According to documents OpenAI distributed to investors, that determination “is in the ‘reasonable discretion’ of the board of OpenAI.”
And there’s the problem: Nobody agrees on what’s being measured, or why. If AI programs are bad at Chollet’s test, maybe it just means that they have a hard time visualizing colorful grids rather than anything deeper. And bots that never solve ARC-AGI-2 could generate $100 billion in profits some day. Any specific test—the LSAT or ARC-AGI or a coding puzzle—will inherently contradict the notion of general intelligence; the term’s defining trait may be its undefinability.
The deeper issue, perhaps, is that human intelligence is poorly understood, and gauging it is an infamously hard and prejudiced task. People have knacks for different things, or might arrive at the same result—the answer to a math problem, the solution to an ARC-AGI grid—via very different routes. A person who scores 30 percent on ARC-AGI-2 is in no sense inferior to someone who scores 90 percent. The collision of those differing routes and minds is what sparks debate, creativity, and beauty. Intentions, emotions, and lived experiences drive people as much as any logical reasoning.
Human cognitive diversity, in other words, is a glorious jumble. How do you even begin to construct an artificial version of that? And when that diversity is already so abundant, do you really want to?