The Future of Algorithmic Trading Driven by Data Mining
Algorithmic trading refers to the use of computer programs that execute trades automatically based on predefined mathematical rules. While this strategy was once reserved for institutional investors, advancements in technology have made it increasingly accessible to individual traders. In particular, the integration of data mining techniques has enhanced the precision and responsiveness of algorithms by enabling real-time analysis of financial data. Today, algorithmic trading has become an essential strategy across various markets, including stocks, futures, and forex. Automated trading systems operate without human intervention, executing orders in real-time based on market conditions. These systems are highly valued for their speed and accuracy in executing trades. In recent years, the combination of artificial intelligence, machine learning, and data mining has moved algorithmic trading beyond simple rule-based operations, allowing for more sophisticated, predictive strategies. These technological advancements are now applied across a range of trading approaches, from short-term price fluctuations to long-term trend analysis, supporting both automated decision-making and improved risk management. The Role and Function of Data Mining What is Data Mining? Data mining refers to the process of discovering meaningful patterns and insights from large datasets. It involves statistical analysis, machine learning, and artificial intelligence techniques to make sense of historical data and predict future trends. In the financial industry, data mining plays a crucial role by analyzing various data types such as market prices, trading volumes, and news, thereby supporting the development of data-driven investment strategies. Extracting Insights from Massive Financial Data The financial market generates millions of transactions per second, along with a vast amount of data including company financials, economic indicators, and global news. Data mining systematically processes and analyzes this complex information to identify valuable patterns or market anomalies. As a result, traders can move beyond intuition-based decisions and adopt a more strategic, data-centric approach to investment. How Data Mining Enhances Algorithmic Trading Strategies In algorithmic trading, data mining plays an essential role in both designing and validating trading strategies. By analyzing historical data, it helps identify recurring price patterns and responses to specific market events, which can be encoded into automated trading algorithms. Furthermore, data mining enables real-time market analysis, allowing trading systems to quickly adapt to changes, thereby improving both the precision and profitability of algorithmic trades. Technologies Driving the Precision of Algorithmic Trading Machine Learning-Based Investment Strategies and the Adoption of Deep Learning In recent years, machine learning has become a key element in developing investment strategies. These algorithms analyze historical prices, volumes, and even news data to predict market movements. Deep learning, in particular, excels at recognizing complex patterns in unstructured data. As a result, it enhances the precision of algorithmic trading beyond traditional analysis methods. Utilization of Data Mining in Quantitative Investing Quantitative investing relies on mathematical models and statistical analysis for decision-making, and data mining plays a central role in this approach. By extracting hidden rules and correlations from vast financial datasets, it increases predictive accuracy and helps uncover new investment opportunities. Data mining also assists in validating and refining existing strategies to ensure greater reliability and sustainability. Refinement of Risk Analysis and Pattern Prediction Risk management and market forecasting in algorithmic trading are becoming increasingly sophisticated. With data mining techniques, traders can analyze historical volatility, trading volumes, and external factors to identify potential risks in advance. Additionally, by learning recurring market patterns, systems can determine more accurate entry and exit points, helping to minimize losses and maximize profitability. The Future of Investment Automation and Its Impact on the Market Expansion of Data-Driven Decision-Making Algorithmic trading is increasingly shifting from intuition-based decisions to those grounded in data analysis. With access to vast datasets including market trends, news, and even social media sentiment trading algorithms can now make more precise and timely decisions. The International Organization of Securities Commissions (IOSCO) has noted that data-driven investment systems contribute significantly to improving market efficiency and transparency. Competition and Collaboration Between Human Traders and Algorithms While traditionally seen as competitors, human traders and algorithmic systems are now more often c
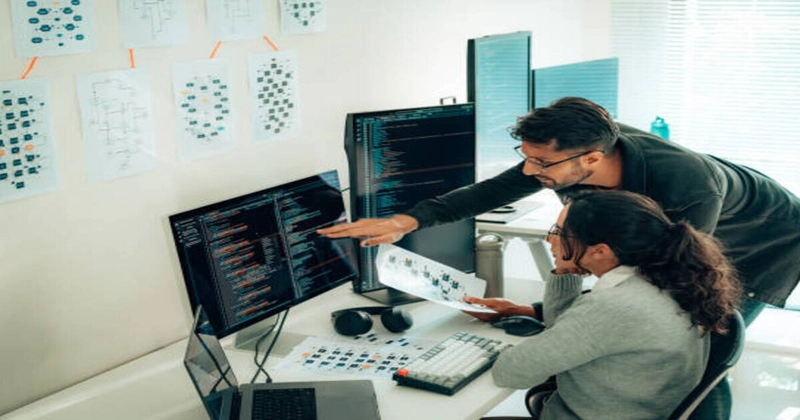
Algorithmic trading refers to the use of computer programs that execute trades automatically based on predefined mathematical rules. While this strategy was once reserved for institutional investors, advancements in technology have made it increasingly accessible to individual traders. In particular, the integration of data mining techniques has enhanced the precision and responsiveness of algorithms by enabling real-time analysis of financial data. Today, algorithmic trading has become an essential strategy across various markets, including stocks, futures, and forex.
Automated trading systems operate without human intervention, executing orders in real-time based on market conditions. These systems are highly valued for their speed and accuracy in executing trades. In recent years, the combination of artificial intelligence, machine learning, and data mining has moved algorithmic trading beyond simple rule-based operations, allowing for more sophisticated, predictive strategies. These technological advancements are now applied across a range of trading approaches, from short-term price fluctuations to long-term trend analysis, supporting both automated decision-making and improved risk management.
The Role and Function of Data Mining
What is Data Mining?
Data mining refers to the process of discovering meaningful patterns and insights from large datasets. It involves statistical analysis, machine learning, and artificial intelligence techniques to make sense of historical data and predict future trends. In the financial industry, data mining plays a crucial role by analyzing various data types such as market prices, trading volumes, and news, thereby supporting the development of data-driven investment strategies.
Extracting Insights from Massive Financial Data
The financial market generates millions of transactions per second, along with a vast amount of data including company financials, economic indicators, and global news. Data mining systematically processes and analyzes this complex information to identify valuable patterns or market anomalies. As a result, traders can move beyond intuition-based decisions and adopt a more strategic, data-centric approach to investment.
How Data Mining Enhances Algorithmic Trading Strategies
In algorithmic trading, data mining plays an essential role in both designing and validating trading strategies. By analyzing historical data, it helps identify recurring price patterns and responses to specific market events, which can be encoded into automated trading algorithms. Furthermore, data mining enables real-time market analysis, allowing trading systems to quickly adapt to changes, thereby improving both the precision and profitability of algorithmic trades.
Technologies Driving the Precision of Algorithmic Trading
Machine Learning-Based Investment Strategies and the Adoption of Deep Learning
In recent years, machine learning has become a key element in developing investment strategies. These algorithms analyze historical prices, volumes, and even news data to predict market movements. Deep learning, in particular, excels at recognizing complex patterns in unstructured data. As a result, it enhances the precision of algorithmic trading beyond traditional analysis methods.
Utilization of Data Mining in Quantitative Investing
Quantitative investing relies on mathematical models and statistical analysis for decision-making, and data mining plays a central role in this approach. By extracting hidden rules and correlations from vast financial datasets, it increases predictive accuracy and helps uncover new investment opportunities. Data mining also assists in validating and refining existing strategies to ensure greater reliability and sustainability.
Refinement of Risk Analysis and Pattern Prediction
Risk management and market forecasting in algorithmic trading are becoming increasingly sophisticated. With data mining techniques, traders can analyze historical volatility, trading volumes, and external factors to identify potential risks in advance. Additionally, by learning recurring market patterns, systems can determine more accurate entry and exit points, helping to minimize losses and maximize profitability.
The Future of Investment Automation and Its Impact on the Market
Expansion of Data-Driven Decision-Making
Algorithmic trading is increasingly shifting from intuition-based decisions to those grounded in data analysis. With access to vast datasets including market trends, news, and even social media sentiment trading algorithms can now make more precise and timely decisions. The International Organization of Securities Commissions (IOSCO) has noted that data-driven investment systems contribute significantly to improving market efficiency and transparency.
Competition and Collaboration Between Human Traders and Algorithms
While traditionally seen as competitors, human traders and algorithmic systems are now more often complementing each other. In hybrid models, algorithms handle rapid data analysis and execution, while human traders apply strategic judgment and oversight. According to the MIT Laboratory for Financial Engineering, such collaborative models have shown strong performance in both profitability and risk management, highlighting the value of human-algorithm synergy.
New Investment Opportunities for Retail Investors
The democratization of algorithmic trading has opened new doors for individual investors. Tools and platforms that were once exclusive to institutional investors are now accessible through fintech services. Retail investors can benefit from automated portfolio management and personalized asset allocation strategies. This shift represents a meaningful step toward broader access to sophisticated investment methods, promoting greater equity in financial markets.
The Next Phase of Algorithmic Trading
Strategies based on data mining are gaining attention as long-term investment tools. Moving beyond simple technical indicators, traders now leverage machine learning and statistical models for deeper analysis. By utilizing diverse market data, including unstructured sources, predictive accuracy improves. However, as markets evolve, continuous validation and periodic rebalancing of algorithms are essential for sustained effectiveness.
Advancements in AI, cloud computing, and high-speed networks are expanding the scope and influence of algorithmic trading. Real-time data analysis and improved trade execution speed enhance efficiency and support strategies such as high-frequency trading. These developments not only increase market liquidity but also influence price discovery mechanisms, prompting a potential restructuring of traditional market operations.
Algorithmic trading was once seen as a strategy exclusive to institutional investors, but its accessibility has grown significantly. The rise of open-source platforms, automation tools, and data analytics has lowered the barrier for individual investors. As a result, algorithmic trading is no longer an auxiliary approach but a core method in modern trading. Its role in financial markets is expected to continue expanding in the coming years.Visit 프리또, where a more stable and enhanced solution is being built.