Balancing Automation and Expertise in Machine Learning with AutoML
Balancing Automation and Expertise in Machine Learning with AutoML As we journey deeper into the age of artificial intelligence, businesses are confronted with a growing demand for data-driven decision-making. The complexities of machine learning, once confined to the realms of data scientists and tech gurus, are now being simplified by a game-changing innovation: Automated Machine Learning, or AutoML. This powerful technology enables anyone regardless of their technical background to build sophisticated machine learning models and extract valuable insights from their data. However, as we embrace this wave of automation, a crucial question arises: how do we maintain the essential balance between the efficiency of automation and the irreplaceable value of human expertise? Let’s explore this dynamic landscape where technology and human insight converge to unlock new possibilities in machine learning. What is Automated Machine Learning? Automated Machine Learning, or AutoML, encompasses a range of techniques and tools designed to automate the process of applying machine learning to real-world challenges. Traditionally, crafting machine learning models required extensive knowledge of algorithms, coding, and statistical analysis. However, with the advent of AutoML, even those without deep technical expertise can successfully create, train, and deploy effective machine learning models. Leading platforms like Google Cloud AutoML, H2O.ai, and DataRobot are revolutionizing this landscape, offering intuitive interfaces and robust features that simplify every step of the model-building process. AutoML has not only democratized machine learning but also significantly reduced the time and resources required to develop effective models. This has opened up opportunities for businesses and organizations of all sizes to leverage the power of machine learning for various applications, from predictive analytics to image recognition. For example, Google Cloud AutoML offers a user-friendly interface that allows users to upload their data, select the type of model they want to build, and then let the platform handle the rest, including hyperparameter tuning and model evaluation. As a result, businesses can now focus on deriving insights from their data and making informed decisions, rather than getting bogged down in the technical complexities of model development. The impact of AutoML is evident in industries ranging from healthcare to finance, where organizations are using these tools to drive innovation and gain a competitive edge. The Benefits of Automated Machine Learning Accessibility: One of the most significant advantages of automated machine learning is its ability to democratize AI. By bridging the gap between complex algorithms and everyday users, AutoML empowers non-experts to engage with machine learning technologies. This newfound accessibility enables a wider range of individuals to contribute to data-driven initiatives within their organizations. Efficiency: Time is often a critical factor in the business world, and automated machine learning can dramatically accelerate the model development process. By automating repetitive tasks such as feature selection, model training, and hyperparameter tuning, AutoML allows users to redirect their focus from technical minutiae to strategic decision-making. This efficiency can lead to faster time-to-market for innovative solutions. Improved Accuracy: The sophistication of automated machine learning algorithms can often surpass the accuracy achieved through manual model-building methods. AutoML platforms leverage advanced techniques to fine-tune parameters and optimize models, resulting in higher predictive performance. This is particularly beneficial for organizations that rely on data-driven insights for critical decision-making. Cost-Effectiveness: The deployment of automated machine learning can lead to significant cost savings. By reducing the dependency on large teams of data scientists, businesses can allocate resources more efficiently while still harnessing the transformative power of machine learning. This is especially valuable for smaller organizations or startups looking to leverage AI without incurring substantial overhead costs. The Human Element: Why Expertise Still Matters While automated machine learning offers remarkable benefits, it is crucial to acknowledge that human expertise remains an indispensable component of the machine learning process. Here’s why the human touch is still vital: Understanding the Business Problem: Human experts bring a wealth of knowledge about the specific challenges and nuances within their industry. They can provide valuable insights that automated systems may overlook, ensuring that the machine learning model aligns with the organization’s business objectives and addresses real-world problems effectively. Data Quality: The age-old adage “garbage in, garbage out” rings true in the context of ma
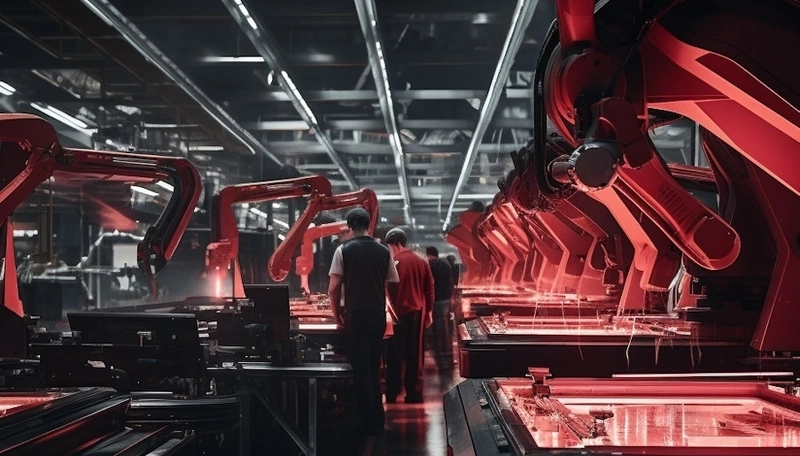
Balancing Automation and Expertise in Machine Learning with AutoML
As we journey deeper into the age of artificial intelligence, businesses are confronted with a growing demand for data-driven decision-making. The complexities of machine learning, once confined to the realms of data scientists and tech gurus, are now being simplified by a game-changing innovation: Automated Machine Learning, or AutoML.
This powerful technology enables anyone regardless of their technical background to build sophisticated machine learning models and extract valuable insights from their data. However, as we embrace this wave of automation, a crucial question arises: how do we maintain the essential balance between the efficiency of automation and the irreplaceable value of human expertise? Let’s explore this dynamic landscape where technology and human insight converge to unlock new possibilities in machine learning.
What is Automated Machine Learning?
Automated Machine Learning, or AutoML, encompasses a range of techniques and tools designed to automate the process of applying machine learning to real-world challenges. Traditionally, crafting machine learning models required extensive knowledge of algorithms, coding, and statistical analysis.
However, with the advent of AutoML, even those without deep technical expertise can successfully create, train, and deploy effective machine learning models. Leading platforms like Google Cloud AutoML, H2O.ai, and DataRobot are revolutionizing this landscape, offering intuitive interfaces and robust features that simplify every step of the model-building process.
AutoML has not only democratized machine learning but also significantly reduced the time and resources required to develop effective models. This has opened up opportunities for businesses and organizations of all sizes to leverage the power of machine learning for various applications, from predictive analytics to image recognition.
For example, Google Cloud AutoML offers a user-friendly interface that allows users to upload their data, select the type of model they want to build, and then let the platform handle the rest, including hyperparameter tuning and model evaluation. As a result, businesses can now focus on deriving insights from their data and making informed decisions, rather than getting bogged down in the technical complexities of model development. The impact of AutoML is evident in industries ranging from healthcare to finance, where organizations are using these tools to drive innovation and gain a competitive edge.
The Benefits of Automated Machine Learning
Accessibility: One of the most significant advantages of automated machine learning is its ability to democratize AI. By bridging the gap between complex algorithms and everyday users, AutoML empowers non-experts to engage with machine learning technologies. This newfound accessibility enables a wider range of individuals to contribute to data-driven initiatives within their organizations.
Efficiency: Time is often a critical factor in the business world, and automated machine learning can dramatically accelerate the model development process. By automating repetitive tasks such as feature selection, model training, and hyperparameter tuning, AutoML allows users to redirect their focus from technical minutiae to strategic decision-making. This efficiency can lead to faster time-to-market for innovative solutions.
Improved Accuracy: The sophistication of automated machine learning algorithms can often surpass the accuracy achieved through manual model-building methods. AutoML platforms leverage advanced techniques to fine-tune parameters and optimize models, resulting in higher predictive performance. This is particularly beneficial for organizations that rely on data-driven insights for critical decision-making.
Cost-Effectiveness: The deployment of automated machine learning can lead to significant cost savings. By reducing the dependency on large teams of data scientists, businesses can allocate resources more efficiently while still harnessing the transformative power of machine learning. This is especially valuable for smaller organizations or startups looking to leverage AI without incurring substantial overhead costs.
The Human Element: Why Expertise Still Matters
While automated machine learning offers remarkable benefits, it is crucial to acknowledge that human expertise remains an indispensable component of the machine learning process. Here’s why the human touch is still vital:
Understanding the Business Problem: Human experts bring a wealth of knowledge about the specific challenges and nuances within their industry. They can provide valuable insights that automated systems may overlook, ensuring that the machine learning model aligns with the organization’s business objectives and addresses real-world problems effectively.
Data Quality: The age-old adage “garbage in, garbage out” rings true in the context of machine learning. The quality of data used for training models is paramount. Data scientists play a crucial role in ensuring that the data is clean, relevant, and representative of the target population. Their expertise is essential for preprocessing data to achieve the best outcomes.
Interpreting Results: Although automated machine learning platforms can create models, interpreting the results and understanding their implications requires a human touch. Experts are needed to provide context, identify potential biases in the data, and make informed decisions based on the outcomes of the model.
Continuous Improvement: Machine learning is not a one-time endeavor; it is an iterative process that requires continuous monitoring and adjustment. Human experts are crucial for evaluating model performance over time and making necessary refinements to ensure ongoing success.
Finding the Right Balance
Achieving a harmonious balance between automation and expertise in the realm of automated machine learning is essential for maximizing its benefits. Here are some strategies to consider:
Collaboration: Foster a culture of collaboration between data scientists and business teams. By working together, they can leverage the strengths of both automation and human insight, leading to more effective and informed decision-making.
Training: Equip non-experts with training on how to effectively utilize AutoML tools. This education empowers individuals to make informed decisions and enhances their understanding of the technology, ultimately leading to better results.
Monitor and Evaluate: Continuously monitor the performance of models generated by automated machine learning platforms. Regularly evaluate their impact on business outcomes to ensure that they remain relevant and effective. This proactive approach helps organizations stay ahead of the curve.
Embrace Adaptation: Be open to refining and improving models over time. Automated machine learning provides a strong foundation, but human expertise is essential for ongoing success. Emphasizing iteration allows for continuous growth and adaptation in a rapidly changing environment.
Closing Note:
Automated machine learning is revolutionizing the landscape of machine learning, making it accessible to a broader audience and streamlining the development process. However, it is vital to remember that human expertise remains irreplaceable. By finding the right balance between automation and expertise, organizations can harness the full potential of automated machine learning to drive growth, innovation, and informed decision-making.
At Levitation, we believe in empowering businesses to effectively leverage technology, and automated machine learning is a powerful tool in that journey. Whether you’re just beginning your AI journey or looking to enhance your existing machine learning capabilities, our team is here to help you navigate the complexities of AI and unlock new possibilities for your business.